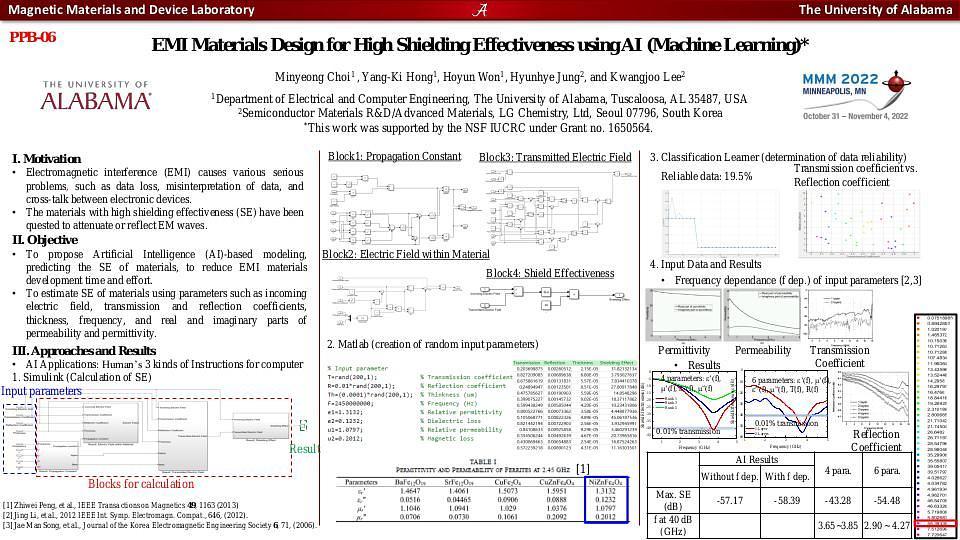
Premium content
Access to this content requires a subscription. You must be a premium user to view this content.
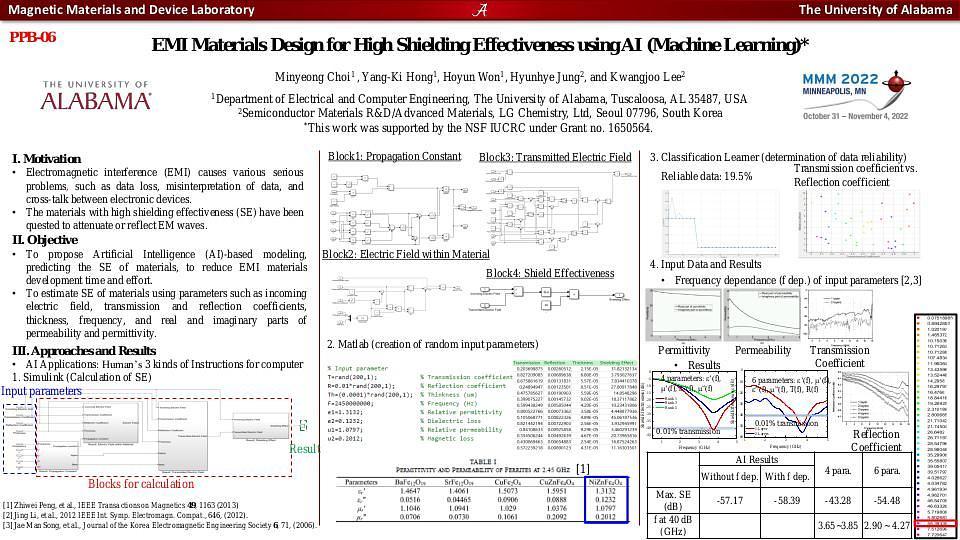
poster
EMI Materials Design for High Shielding Effectiveness using AI (Machine Learning)
Electromagnetic interference (EMI) causes various serious problems such as data loss, misinterpretation of data, and cross-talk between electronic devices. Thus, the
materials with high shielding effectiveness (SE) have been quested to attenuate or reflect EM waves. Various materials and compositions and synthetic methods have been investigated,
optimized, and adapted to achieve the desired SE (> 30 dB) 1-4. These approaches can provide the optimal SE of the material of interest but are costly due to a long period of optimization
and expensive characterization of investigated materials.
To reduce EMI materials development time and effort, we propose Artificial Intelligence (AI)-based modeling, predicting the SE of materials. We used MATLAB to create random input
parameters, Simulink to calculate SE, and Classification Learner to determine data reliability. We take the following nine input parameters to estimate SE of FINEMET (Fe-Si-Nb-Cu-BCr-
Mn-Ni-Co), MnZn-ferrite, and NiZn-ferrite: incoming electric field, transmission (TC) and reflection (RC) coefficients, thickness, frequency, and real and imaginary parts of
permeability (μr) and permittivity (εr). Fig. 1 shows the Simulink block diagram for calculating SE. First, we sampled 200 random TC and RC of the materials and then calculate the SEs
with seven fixed input parameters. Among calculated SEs (Fig. 2e), the reliable SEs (Fig. 2f) are determined using AI. Our preliminary results show that the reliability was 19.5%, meaning
that 39 of 200 samples are reliable. The estimated SE was compared with the experimental SE 1 to assess the accuracy of the developed AI modeling. The estimated and experimental
maximum SEs are 31.87 dB and 30.61 dB, respectively, as shown in Fig. 2 (d,f,g). Accordingly, the effectiveness of our proposed method is validated. We will present how to predict the
SE of EMI materials in detail.
*This work was supported in part by the National Science Foundation (NSF) IUCRC under Grant No. 2137275.
References:
1 Anatoly B. Rinkevich, Dmitry V Perov, and Yuriy I Ryabkov, Materials, 14, 3499 (2021).
2 Isa ARAZ, Turkish Journal of Electrical Engineering & Computer Sciences, 26, 2996 (2018).
3 Jing Li, yao-Jiang Zhang, Aleksandr Gafarov, Soumya De, Marina Y. Koledintseva, Joel Marchand, David Hess, Todd Durant, Eric Nickerson, James L. Drewniak, and Jun Fan, 2012
IEEE International Symposium on Electromagnetic Compatibility, 646, (2012).
4 Jae Man Song, Dong Il Kim, and Kyeung Jin O, Journal of the Korea Electromagnetic Engineering Society 6, 71, (2006).