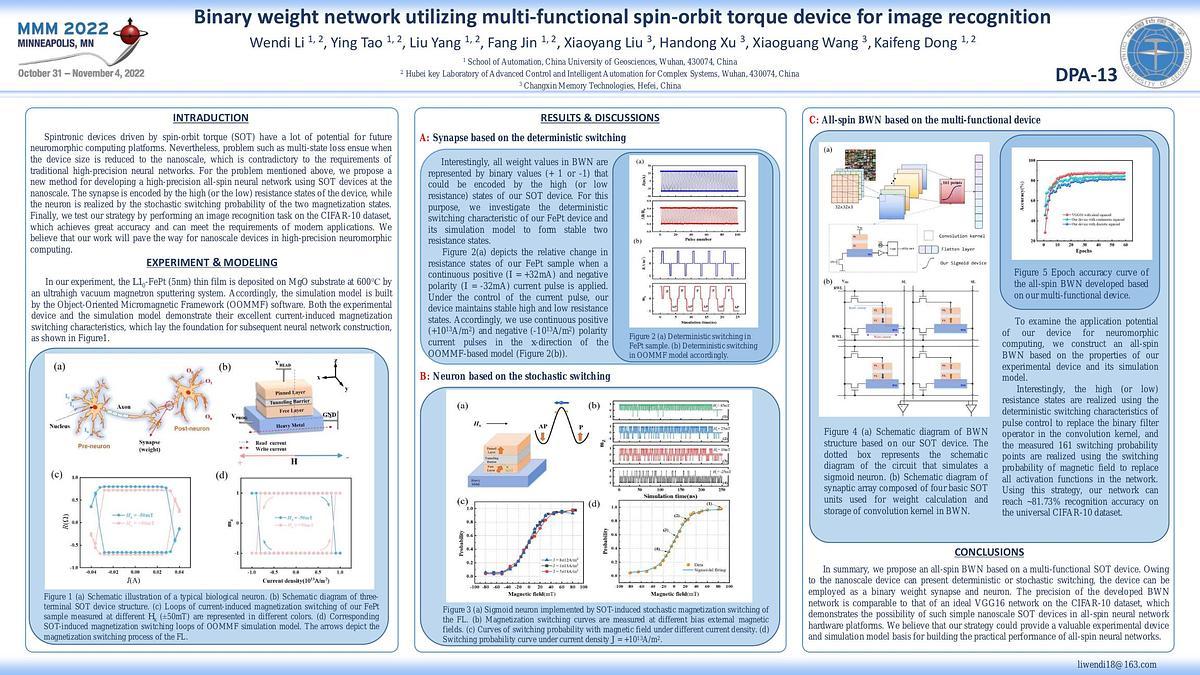
Premium content
Access to this content requires a subscription. You must be a premium user to view this content.
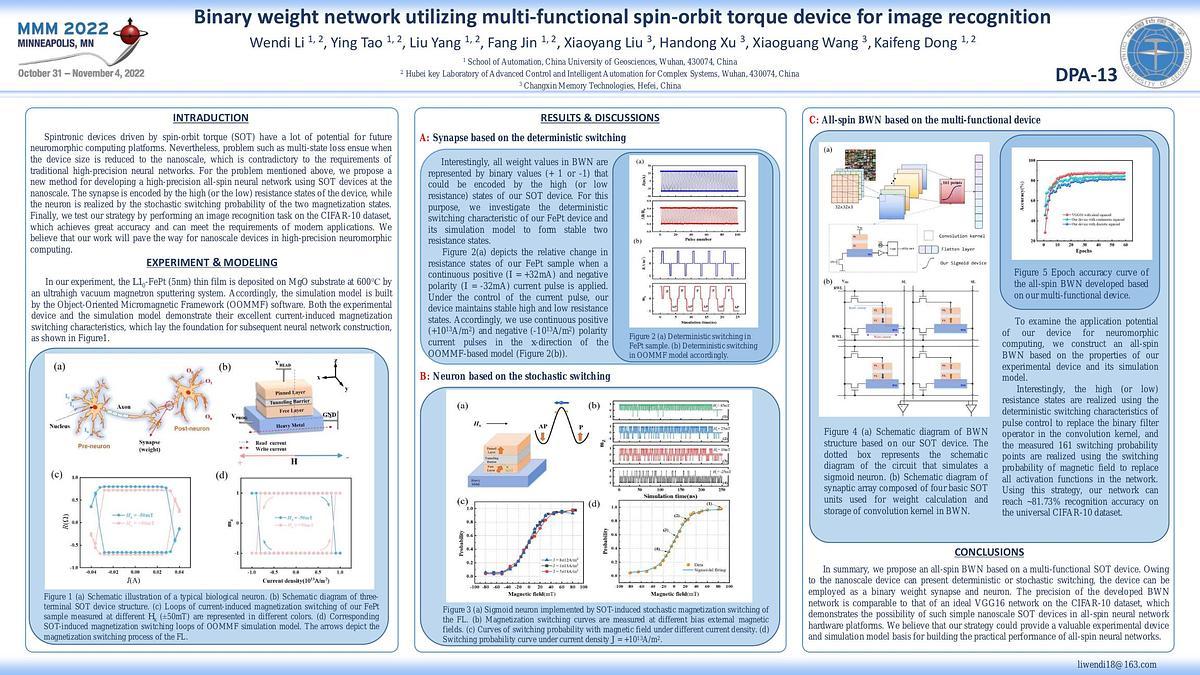
poster
Binary weight network utilizing multi functional spin
Spintronic devices driven by spin-orbit torque (SOT) have a lot of potential for future neuromorphic computing hardware platforms. Nevertheless, problems such as multistate loss, weight time-dependent variability, and output discontinuity ensue when the device size is reduced to the nanoscale, which is contradictory to the needs of traditional high-precision neural networks. In this study, we propose a high-precision all-spin neural network based on magnetization switching of a simple nanoscale multi-functional SOT device. The all-spin neural network is implemented by the Binary Weight Network, in which binary synapse is encoded by the two states formed by the device's deterministic switching and the practical neuron is realized by its stochastic switching probability. Furthermore, we use a difference derivation training algorithm for the general-purpose network in a novel way to be more compatible with the discontinuous neuron output. Using this strategy, our network recognition accuracy can reach ~81.73% on the universal CIFAR-10 dataset, paving the way for the practical hardware implementation of nanoscale SOT devices in high-precision compact neuromorphic computing.
References:
J. Zhou, T. Zhao and J. Chen, Advanced Materials., Vol. 33, p.2103672 (2021)
S. Zhang, J. Zhang and L.You, Science China Information Sciences., Vol. 65, p.1-9 (2022)