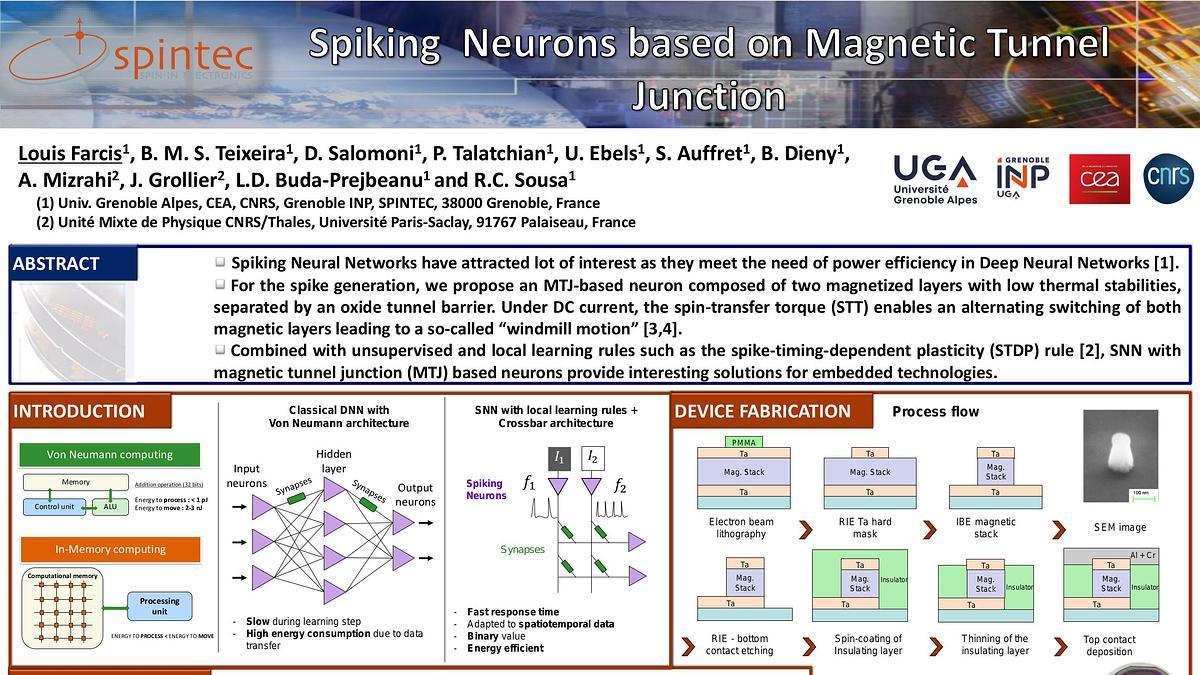
Premium content
Access to this content requires a subscription. You must be a premium user to view this content.
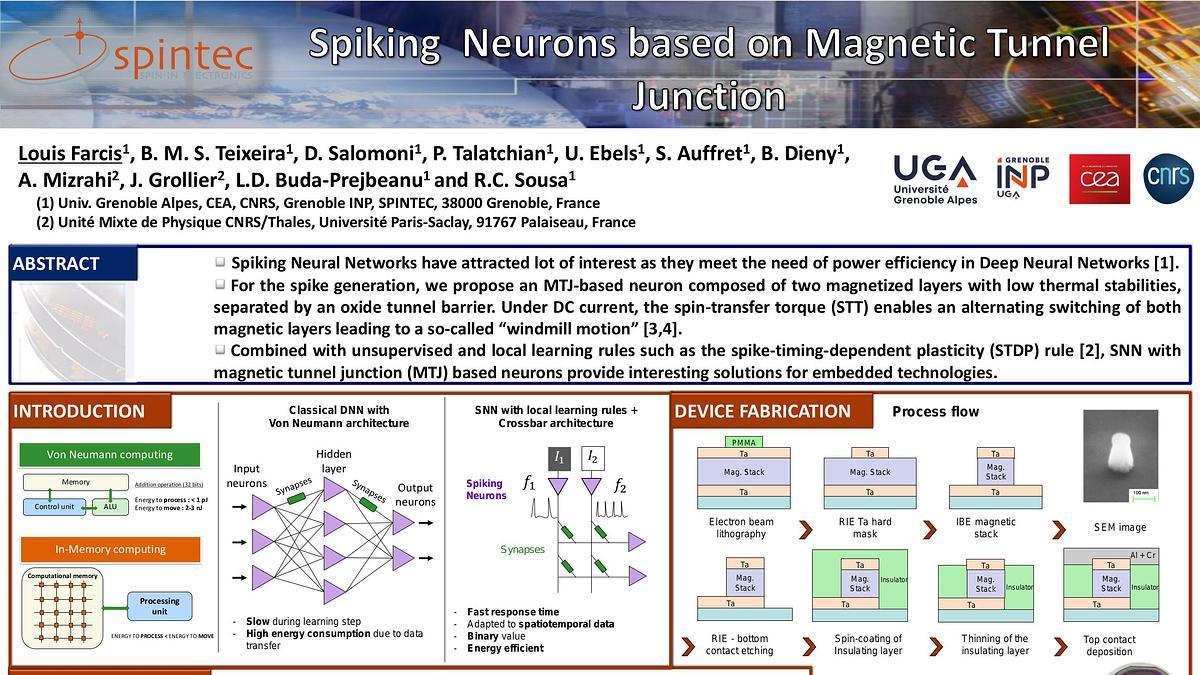
poster
Spiking dynamics with dual free layer MTJ
Spiking neural networks have attracted a lot of interest in the past decade as they meet the need of energy efficiency in deep neural network computing (1). Using spikes instead of continuous signals enables low power operation, event-based computations and fast response time, which is better adapted to spatial-temporal data. Combined with unsupervised and local learning rules such as the spike-timing-dependant plasticity rule (2), SNN with magnetic tunnel junction (MTJ) based neurons provide interesting solutions for embedded technologies with better back-end-of-line compatibility (3) compared to other technologies.
For the spike generation, we propose an MTJ-based neuron composed of two magnetized layers (M1 and M2), with low thermal stabilities, separated by an oxide tunnel barrier. Under applied current, the spin-transfer torque (STT) enables an alternating switching of both magnetic layers leading to a so-called “windmill motion” (see Fig1.a-c) previously predicted by simulation for in-plane and out-of-plane magnetized MTJs (4,5). Here we designed, fabricated and characterized such type of structure and were able to achieve steady alternating magnetization reversal of both layers generating a characteristic spike-like resistive response.
MTJ stacks of FeCoB/MgO/FeCoB, with varying FeCoB thicknesses, were deposited by magnetron sputtering and patterned into nano-pillars with diameters ranging from 50 to 200 nm. The electrical characterization of the nano-pillars reveals essential changes of the magnetization dynamics depending on the pillar diameters, layer thicknesses, voltage, applied magnetic field and temperature of the junction. As predicted by the simulation, the frequency of the oscillations and the width of the spikes evolve with the voltage amplitude and the applied field. Important similarities confirm the parameter dependence with temperature and bias voltage. Controlling the spiking rates at low voltages is a critical requirement to build an efficient spiking neural network.
References Acknowledgements:
This work was supported by ANR via grant SpinSpike Projet-ANR-20-CE24-0002.
References:
(1) A. Tavanaei, M. Ghodrati and S.R. Kheradpisheh, Neural networks, 111, 47-63 (2019).
(2) T. Iakymchuk, A. Rosado-Muñoz and J.F. Guerrero-Martínez, EURASIP Journal on Image and Video Processing 2015, 1-11 (2015).
(3) D. Shum, D. Houssameddine and S.T. Woo, 2017 Symposium on VLSI Technology. IEEE pp. T208-T209 (2017).
(4) R. Matsumoto, S. Lequeux, and J. Grollier, Physical Review Applied, 11(4), 044093 (2019)
(5) G. Gupta, Z. Zhu and G. Liang, arXiv preprint arXiv, 1611.05169 (2016).