
Sewon Min
Graduate student @ University of Washington
in-context learning
question answering
pragmatics
reasoning
few-shot learning
dialogue systems
language modeling
large language models
open-domain question answering
chain-of-thought
natural language understanding
information-seeking
11
presentations
155
number of views
SHORT BIO
Sewon Min is a Ph.D. candidate in the Natural Language Processing group at the University of Washington. She is advised by Hannaneh Hajishirzi and Luke Zettlemoyer. She is supported by a J.P. Morgan PhD Fellowship. She has been a part-time visiting researcher at Meta AI (formerly Facebook AI Research) during her Ph.D., and previously interned at Google Research (in 2020) and Salesforce Research (in 2017). Prior to UW, she received B.S. in CSE from Seoul National University.
Presentations

Towards Understanding Chain-of-Thought Prompting: An Empirical Study of What Matters
Boshi Wang and 6 other authors

Z-ICL: Zero-Shot In-Context Learning with Pseudo-Demonstrations
Xinxi Lyu and 4 other authors
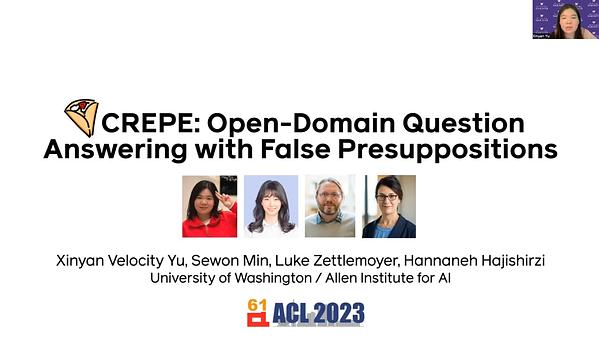
CREPE: Open-Domain Question Answering with False Presuppositions
Xinyan Velocity Yu and 3 other authors

INSCIT: Information-Seeking Conversations with Mixed-Initiative Interactions
Zeqiu Wu and 6 other authors
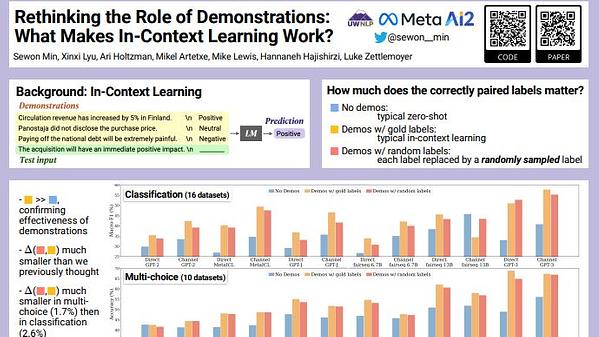
Rethinking the Role of Demonstrations: What Makes In-Context Learning Work?
Sewon Min and 6 other authors
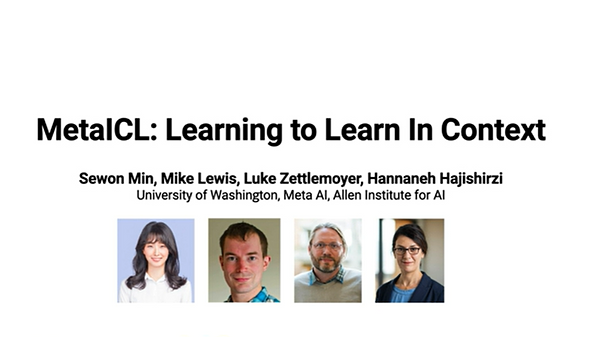
MetaICL: Learning to Learn In Context
Sewon Min and 3 other authors
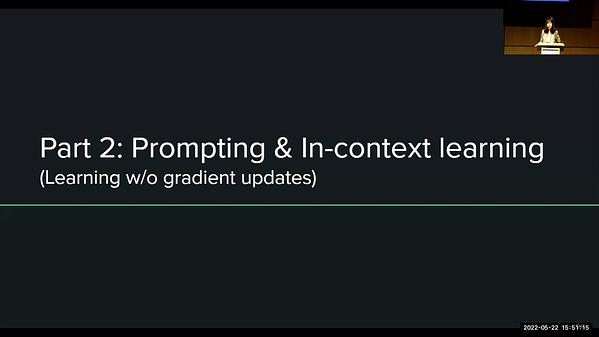
Zero- and Few-Shot NLP with Pretrained Language Models - Prompting & In-context learning
Sewon Min
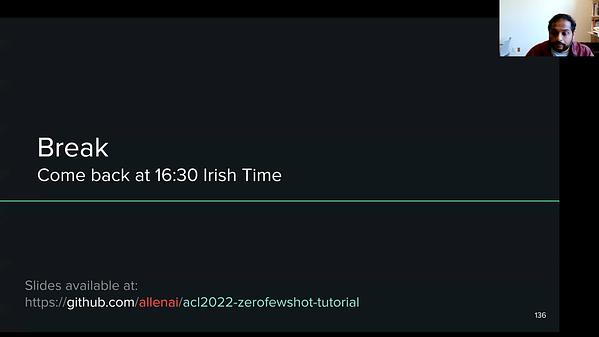
Zero- and Few-Shot NLP with Pretrained Language Models Part 1
Arman Cohan and 4 other authors
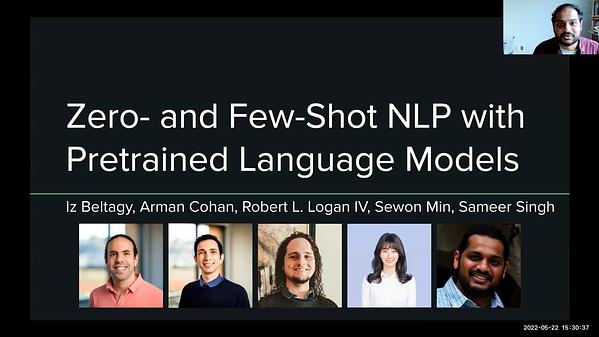
Zero- and Few-Shot NLP with Pretrained Language Models Part 2
Arman Cohan and 4 other authors

Joint Passage Ranking for Diverse Multi-Answer Retrieval
Sewon Min and 4 other authors
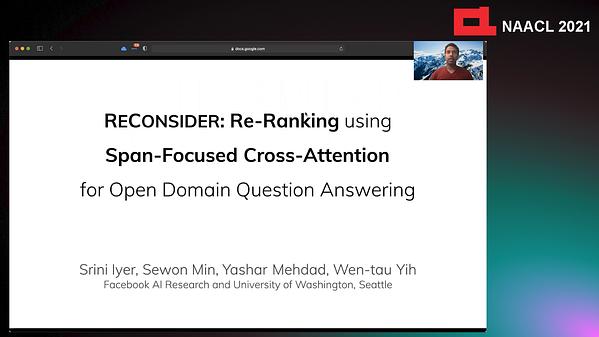
RECONSIDER: Improved Re-Ranking using Span-Focused Cross-Attention for Open Domain Question Answering
Srinivasan Iyer and 3 other authors