
6
presentations
8
number of views
SHORT BIO
I am a final-year Ph.D. student at the University of Illinois, Chicago. My research studies how to make the knowledge in foundation models, particularly large language models (LLMs), more reusable and updatable. This includes (but not limited to) (1) Large Language Model (pre-training, post-training and frontiers, e.g., retrieval-augmented LLM) - presented at ICLR23, EMNLP22a EMNLP22b; (2) Continual and Lifelong Learning (task, class, and domain-incremental) - presented at ICML23, NeurIPS20,21,22; (3) Natural Language Processing (classification, generation and extraction) - presented at EMNLP23, NAACL21, EMNLP21; (4) Argument Mining - presented at ACL18,19; IJCAI18,19.
Presentations
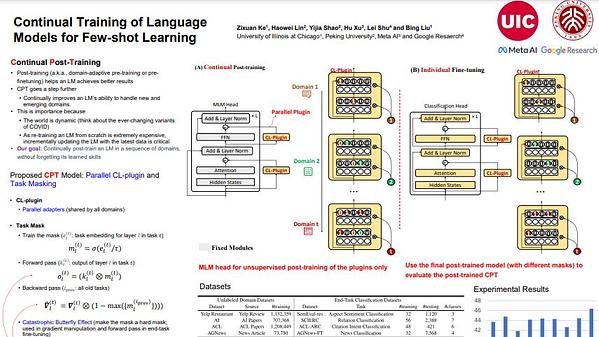
Continual Training of Language Models for Few-Shot Learning
Zixuan Ke
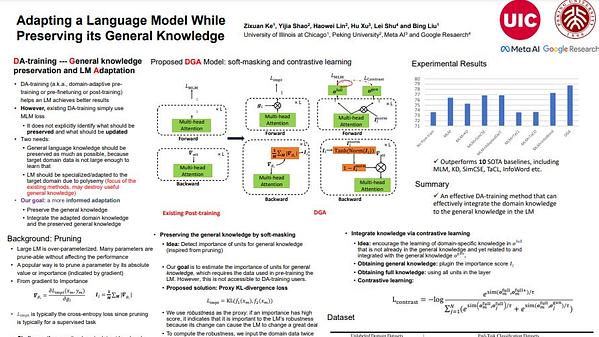
Adapting a Language Model While Preserving its General Knowledge
Zixuan Ke
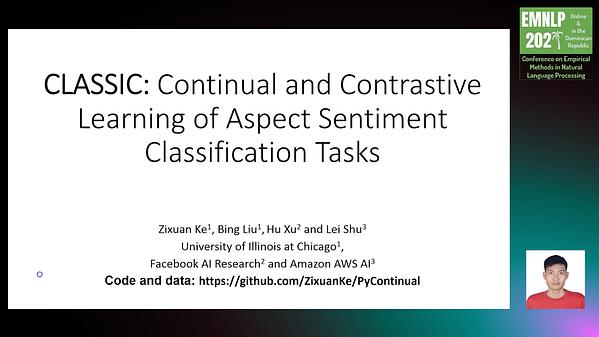
CLASSIC: Continual and Contrastive Learning of Aspect Sentiment Classification Tasks
Zixuan Ke and 3 other authors

CLASSIC: Continual and Contrastive Learning of Aspect Sentiment Classification Tasks
Zixuan Ke and 3 other authors
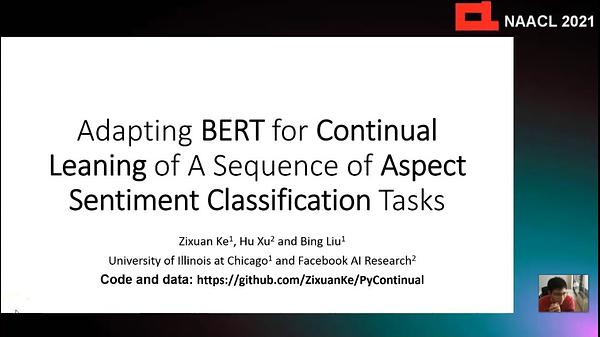
Adapting BERT for Continual Learning of a Sequence of Aspect Sentiment Classification Tasks
Zixuan Ke and 2 other authors