
Christopher D. Manning
Stanford University
question answering
language models
meta-learning
multi-hop question answering
pre-training
compositional generalization
information extraction
few-shot learning
dialogue systems
interpretability
visual question answering
language modeling
knowledge
large language models
active learning
13
presentations
18
number of views
SHORT BIO
Christopher Manning is the inaugural Thomas M. Siebel Professor in Machine Learning in the Departments of Linguistics and Computer Science at Stanford University, Director of the Stanford Artificial Intelligence Laboratory (SAIL), and an Associate Director of the Stanford Human-Centered Artificial Intelligence Institute (HAI). His research goal is computers that can intelligently process, understand, and generate human language material. Manning is a leader in applying Deep Learning to Natural Language Processing, with well-known research on the GloVe model of word vectors, question answering, tree-recursive neural networks, machine reasoning, neural network dependency parsing, neural machine translation, sentiment analysis, and deep language understanding. He also focuses on computational linguistic approaches to parsing, natural language inference, and multilingual language processing, including being a principal developer of Stanford Dependencies and Universal Dependencies. Manning has coauthored leading textbooks on statistical approaches to Natural Language Processing (NLP) (Manning and Schütze 1999) and information retrieval (Manning, Raghavan, and Schütze, 2008), as well as linguistic monographs on ergativity and complex predicates. He is an ACM Fellow, an AAAI Fellow, and an ACL Fellow, and a Past President of the ACL (2015). His research has won ACL, Coling, EMNLP, and CHI Best Paper Awards. He has a B.A. (Hons) from The Australian National University and a Ph.D. from Stanford in 1994, and he held faculty positions at Carnegie Mellon University and the University of Sydney before returning to Stanford. He is the founder of the Stanford NLP group (@stanfordnlp) and manages the development of the Stanford CoreNLP software.
Presentations
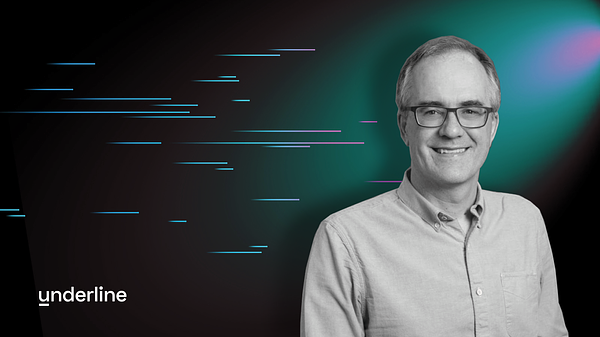
Test of Time Awards
Lilian Lee and 3 other authors

Meta-Learning Online Adaptation of Language Models | VIDEO
Nathan Zixia Hu and 3 other authors
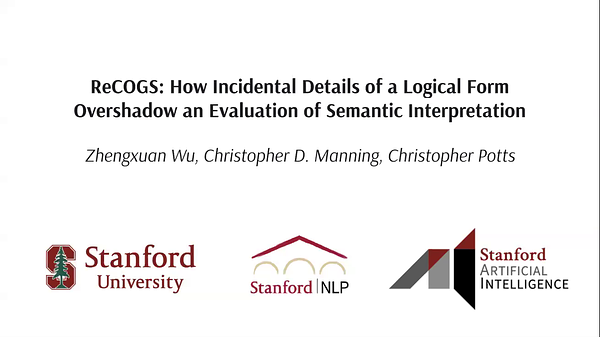
ReCOGS: How Incidental Details of a Logical Form Overshadow an Evaluation of Semantic Interpretation
Zhengxuan Wu and 2 other authors
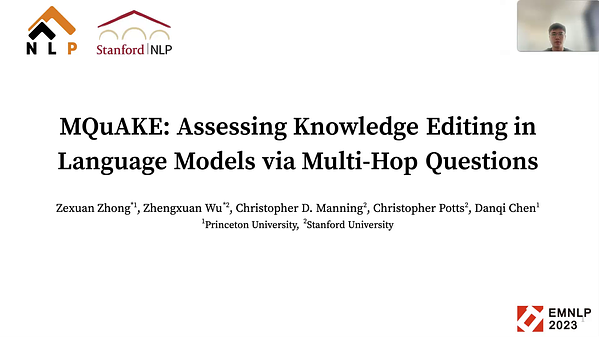
MQuAKE: Assessing Knowledge Editing in Language Models via Multi-Hop Questions
Zexuan Zhong and 4 other authors
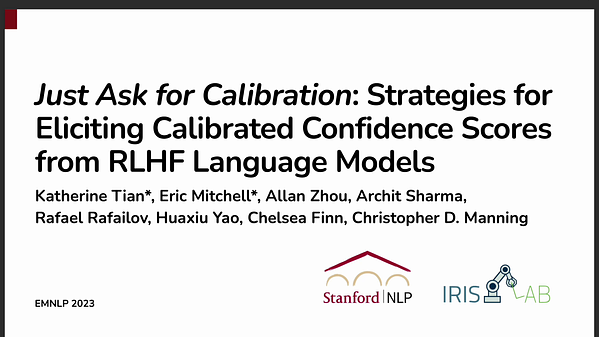
Just Ask for Calibration: Strategies for Eliciting Calibrated Confidence Scores from Language Models Fine-Tuned with Human Feedback
Katherine Tian and 7 other authors

PragmatiCQA: A Dataset for Pragmatic Question Answering in Conversations
Peng Qi and 3 other authors
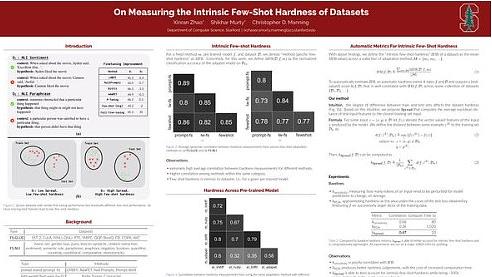
On Measuring the Intrinsic Few-Shot Hardness of Datasets
Xinran Zhao and 2 other authors
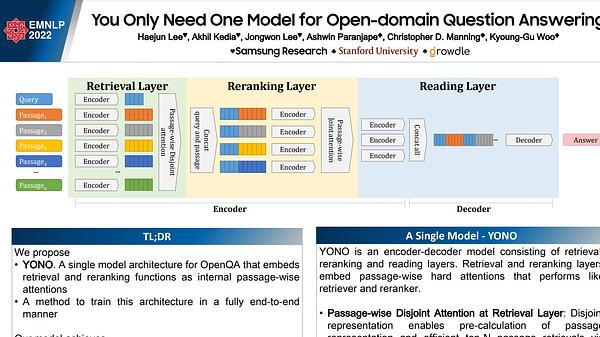
You Only Need One Model for Open-domain Question Answering
Haejun Lee and 5 other authors
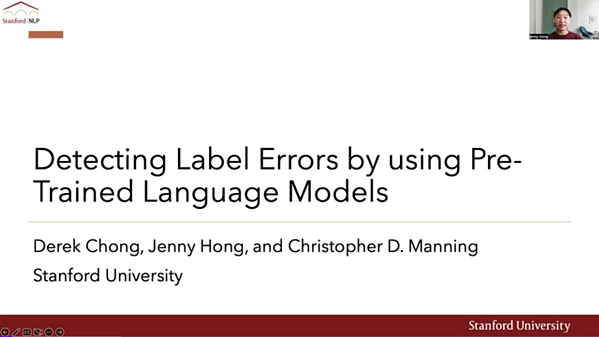
Detecting Label Errors by Using Pre-Trained Language Models
Derek Chong and 2 other authors

Conditional probing: measuring usable information beyond a baseline
John Hewitt and 3 other authors

Conditional probing: measuring usable information beyond a baseline
John Hewitt and 3 other authors
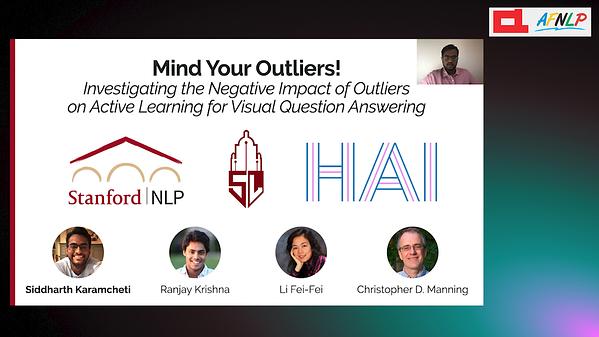
Mind Your Outliers! Investigating the Negative Impact of Outliers on Active Learning for Visual Question Answering
Siddharth Karamcheti and 3 other authors
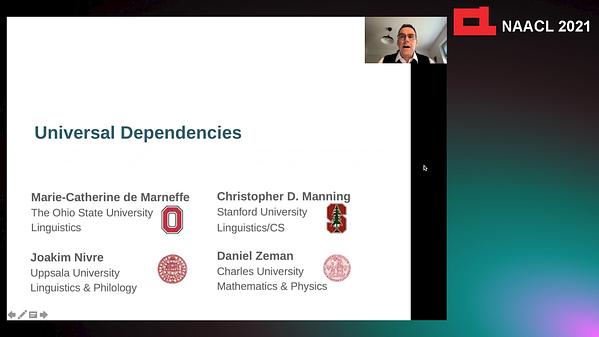
Universal Dependencies
Marie-Catherine de Marneffe and 3 other authors