
Chenglei Si
University of Maryland
pretrained language models
adversarial attack
adversarial robustness
robustness
question answering
pre-trained language model
chinese nlp
fact-checking
large language models
inductive biases
tokenization
code generation
prompting
explanation
calibration
8
presentations
22
number of views
SHORT BIO
I am an Undergrad at UMD CLIP & LSC and an incoming PhD at Stanford NLP. I am advised by Jordan Boyd-Graber at the University of Maryland, while also working closely with Hal Daumé III, He He, Danqi Chen, and Sherry Wu. In summer 2022, I did a research internship at Microsoft hosted by Zhe Gan. Before that, I got into NLP research by working with Min-Yen Kan and Zhiyuan Liu.
Presentations

Large Language Models Help Humans Verify Truthfulness – Except When They Are Convincingly Wrong
Chenglei Si and 6 other authors

Ignore This Title and HackAPrompt: Exposing Systemic Vulnerabilities of LLMs Through a Global Prompt Hacking Competition
Sander V Schulhoff and 9 other authors

Sub-Character Tokenization for Chinese Pretrained Language Models
Chenglei Si
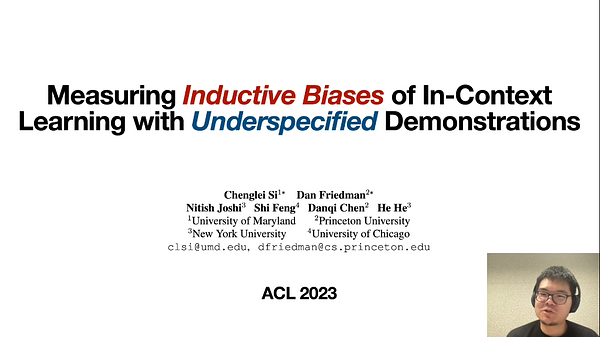
Measuring Inductive Biases of In-Context Learning with Underspecified Demonstrations
Chenglei Si and 5 other authors
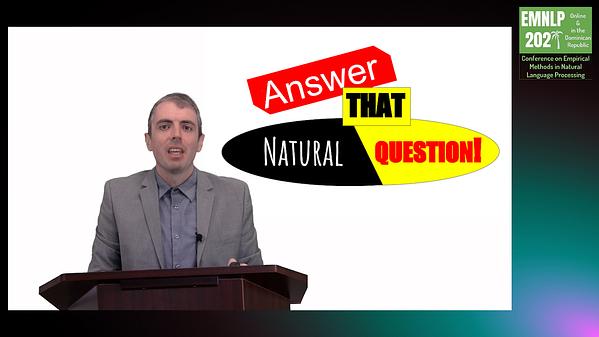
What's in a Name? Answer Equivalence For Open-Domain Question Answering
Chenglei Si and 2 other authors
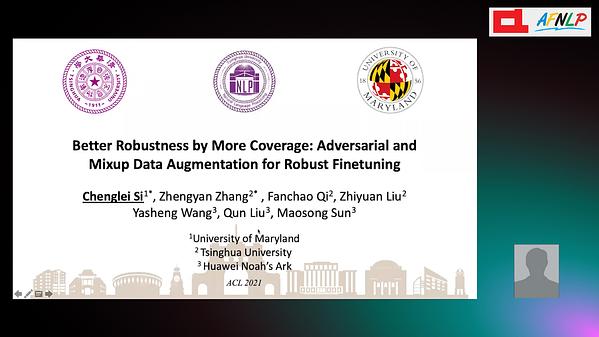
Better Robustness by More Coverage: Adversarial and Mixup Data Augmentation for Robust Finetuning
Chenglei Si and 2 other authors
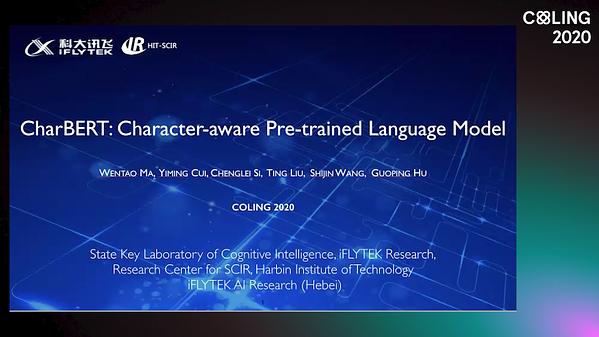
CharBERT: Character-aware Pre-trained Language Model
Wentao Ma and 2 other authors
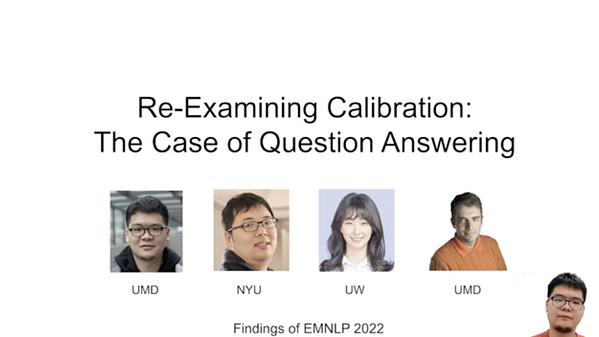
Re-Examining Calibration: The Case of Question Answering
Chenglei Si