
Sijia Liu
adversarial robustness
attack
robustness
privacy
reasoning
deep learning
benchmarking
verification
large language models
interpretation
synthetic data
defense
trustworthy ai
cv
in-context learning
11
presentations
SHORT BIO
Sijia Liu is currently an Assistant Professor at the CSE department of Michigan State University, and an Affiliated Professor at the MIT-IBM Wat- son AI Lab, IBM Research. His research spans the areas of machine learning, optimization, computer vision, signal pro- cessing and computational biology, with a focus on develop- ing learning algorithms and theories for scalable and trustworthy AI. He received the Best Paper Runner-Up Award at the Conference on Uncertainty in Artificial Intelligence (UAI), in 2022. He also received the Best Student Paper Award at the 42nd IEEE International Conference on Acoustics, Speech and Signal Processing (ICASSP), 2017.
Presentations
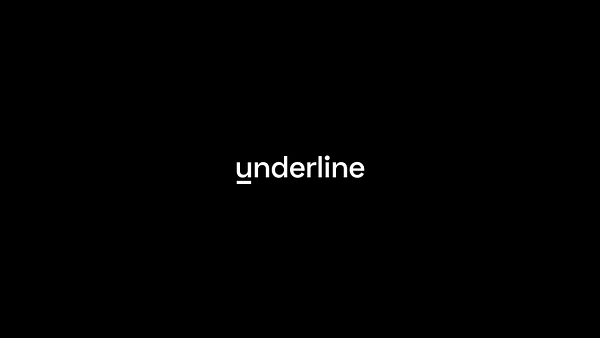
Visual Prompting Upgrades Neural Network Sparsification: A Data-Model Perspective
Can Jin and 6 other authors

SOUL: Unlocking the Power of Second-Order Optimization for LLM Unlearning
Jinghan Jia and 7 other authors

More Samples or More Prompts? Exploring Effective Few-Shot In-Context Learning for LLMs with In-Context Sampling
Bingsheng Yao and 9 other authors

Advancing the Robustness of Large Language Models through Self-Denoised Smoothing
Jiabao Ji and 9 other authors
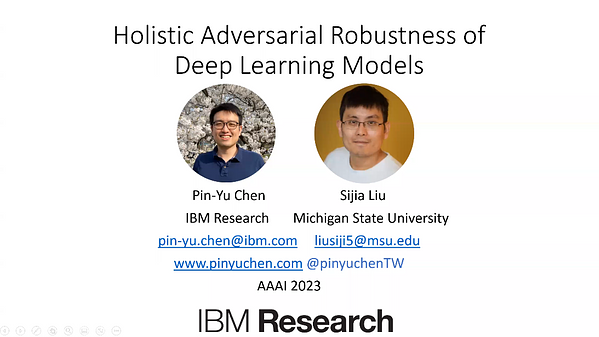
Holistic Adversarial Robustness of Deep Learning Models
Pin-Yu Chen and 1 other author
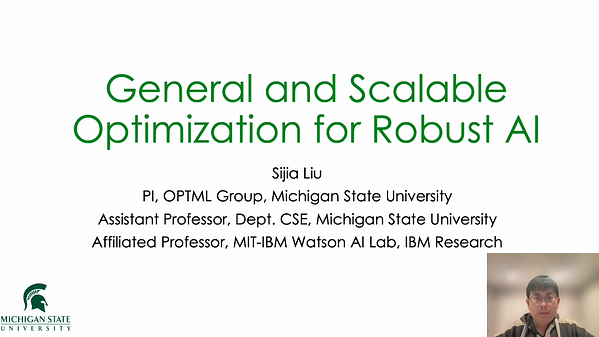
General and Scalable Optimization for Robust AI
Sijia Liu
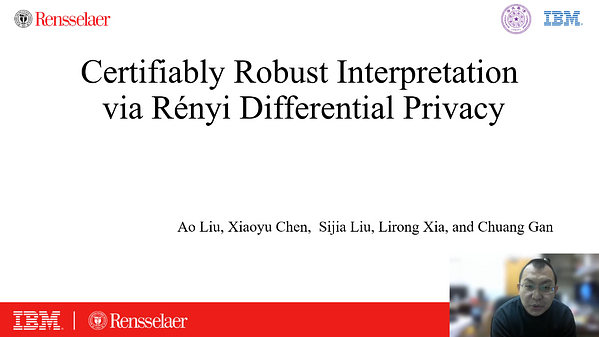
Certifiably Robust Interpretation via Renyi Differential Privacy
Ao Liu and 4 other authors
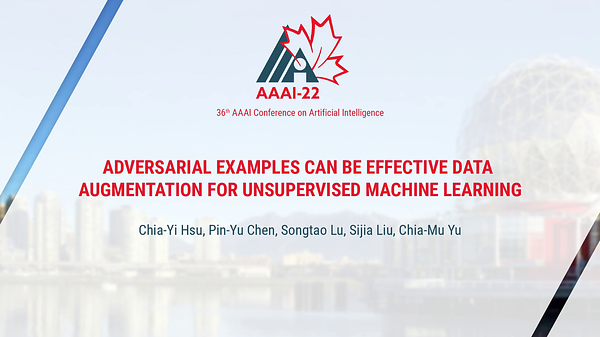
Adversarial Examples can be Effective Data Augmentation for Unsupervised Machine Learning
Chia-Yi Hsu and 4 other authors
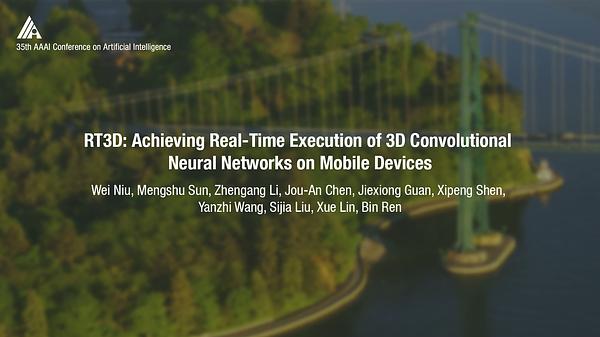
RT3D: Achieving Real-Time Execution of 3D Convolutional Neural Networks on Mobile Devices
Wei Niu and 9 other authors
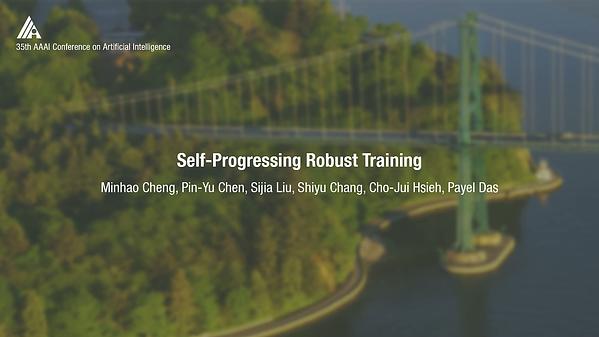
Self-Progressing Robust Training
Minhao Cheng and 5 other authors
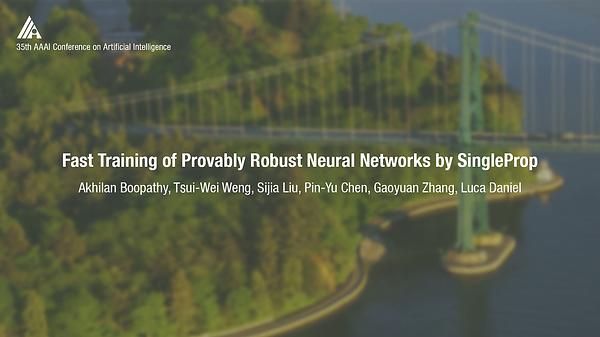
Fast Training of Provably Robust Neural Networks by SingleProp
Akhilan Boopathy and 5 other authors