
2
presentations
1
number of views
SHORT BIO
I am a final year PhD student with an interdisciplinary background who excels in developing and driving my project ideas at the intersection of cognitive science, natural language processing, and computer vision. I have a strong track record of collaborating across research domains in academia as well as industry where I recently completed an internship at Meta. Throughout my PhD, I have focused on implementing cognitively-inspired deep learning methods for language processing and vision/multi-modal interactive applications. I have made substantial contributions to the AI-field, publishing at top tier venues such as NeurIPS, ICCV, CoNLL, and most recently CogSci, by pioneering methods that connect computational models of cognition with deep learning approaches.
I have demonstrated that cognitive models of visual attention can serve as a valuable inductive bias for deep learning models of visual attention - neural networks that predict human-like attention via saliency maps or scanpaths for reading and scene perception. Moreover, by integrating human-like attention into downstream DNNs - such as text summarization and visual question answering - I have achieved state-of-the-art performance across several benchmark datasets while also maintaining computational efficiency. Additionally, my research in explainable AI, is among the first to propose leveraging human visual attention (via eye-tracking data) to interpret learned neural attentive strategies in popular architectures, such as transformer networks.
Presentations

Improving neural saliency prediction with a cognitive model of human visual attention
Ekta Sood and 5 other authors
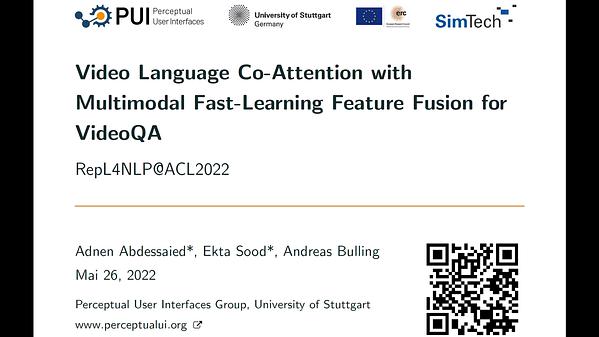
Video Language Co-Attention with Multimodal Fast-Learning Feature Fusion for VideoQA
Adnen Abdessaied and 2 other authors