
Amir Feder
Post-graduate student @ Columbia University
causal inference
low resource
hate speech
interpretability
multimodality
consistency
survey
controllable generation
ontology
human behavior analysis
probability
masked language modeling
model explanations
causal explanations
medical nlp
6
presentations
18
number of views
SHORT BIO
Amir Feder is a postdoctoral fellow at the Columbia Data Science Institute, working with David Blei on causal inference and natural language processing. His research seeks to develop methods that integrate causality into natural language processing, and use them to build linguistically-informed algorithms for predicting and understanding human behavior. Through the paradigm of causal machine learning, Amir aims to build bridges between machine learning and the social sciences.
Before joining Columbia, Amir received his PhD from the Technion, where he was advised by Roi Reichart and worked closely with Uri Shalit. In a previous (academic) life, Amir was an economics, statistics and history student at Tel Aviv University, the Hebrew University of Jerusalem and Northwestern University. Amir was a co-organizer of the First Workshop on Causal Inference and NLP (CI+NLP) at EMNLP 2021.
Presentations

Exploring the Learning Capabilities of Language Models using LEVERWORLDS
Eitan Wagner and 2 other authors
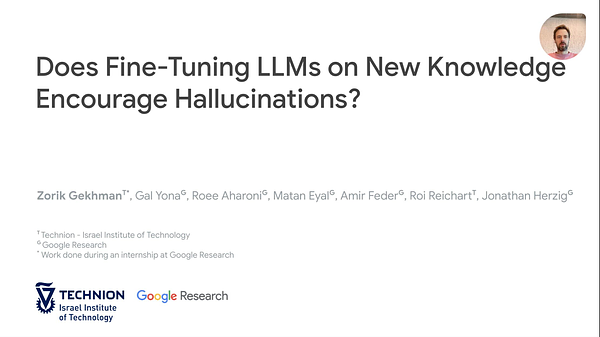
Does Fine-Tuning LLMs on New Knowledge Encourage Hallucinations?
Zorik Gekhman and 6 other authors
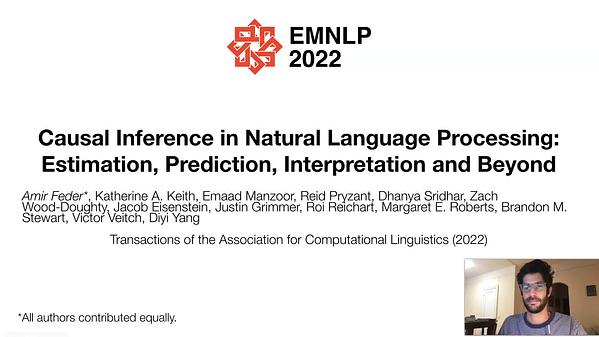
Causal Inference in Natural Language Processing: Estimation, Prediction, Interpretation and Beyond
Amir Feder

Building a Clinically-Focused Problem List From Medical Notes
Amir Feder

DoCoGen: Domain Counterfactual Generation for Low Resource Domain Adaptation
Nitay Calderon and 3 other authors

CausaLM: Causal Model Explanation Through Counterfactual Language Models
Amir Feder and 3 other authors