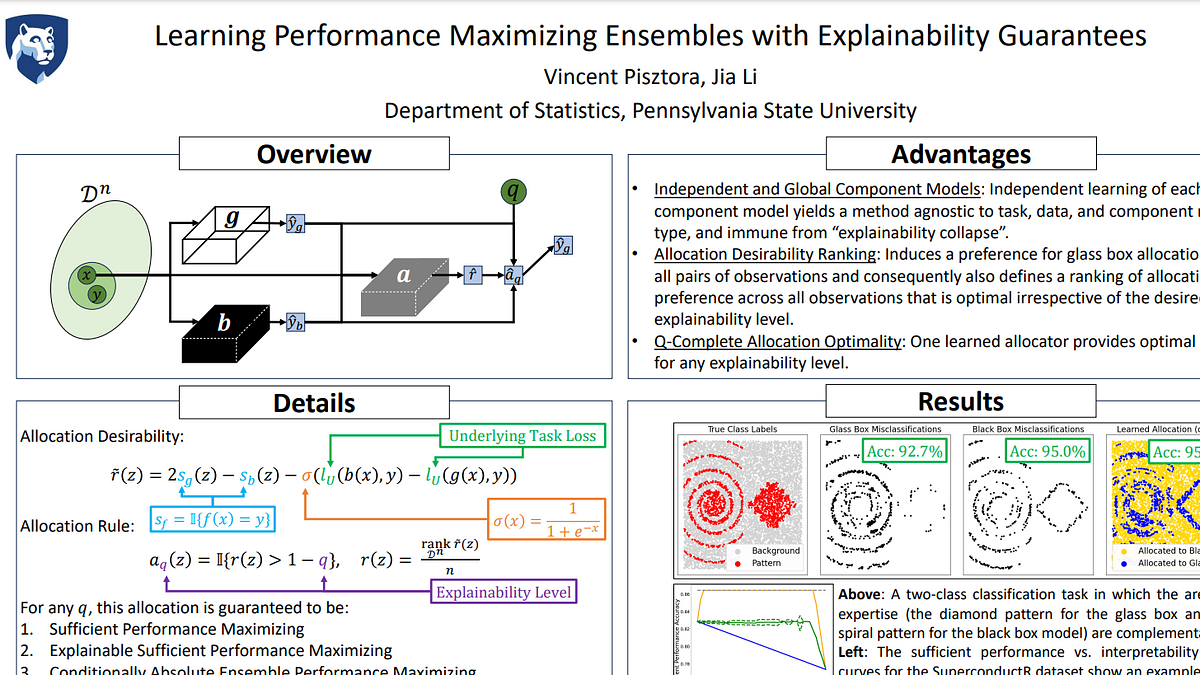
Content not yet available
This lecture has no active video or poster.
technical paper
Learning Performance Maximizing Ensembles with Explainability Guarantees
keywords:
transparent interpretable explainable ml
learning preferences or rankings
ensemble methods
In this paper we propose a method for the optimal allocation of observations between an intrinsically explainable glass box model and a black box model. An optimal allocation being defined as one which, for any given explainability level (i.e. the proportion of observations for which the explainable model is the prediction function), maximizes the performance of the ensemble on the underlying task, and maximizes performance of the explainable model on the observations allocated to it, subject to the maximal ensemble performance condition. The proposed method is shown to produce such explainability optimal allocations on a benchmark suite of tabular datasets across a variety of explainable and black box model types. These learned allocations are found to consistently maintain ensemble performance at very high explainability levels (explaining 74% of observations on average), and in some cases even outperform both the component explainable and black box models while improving explainability.