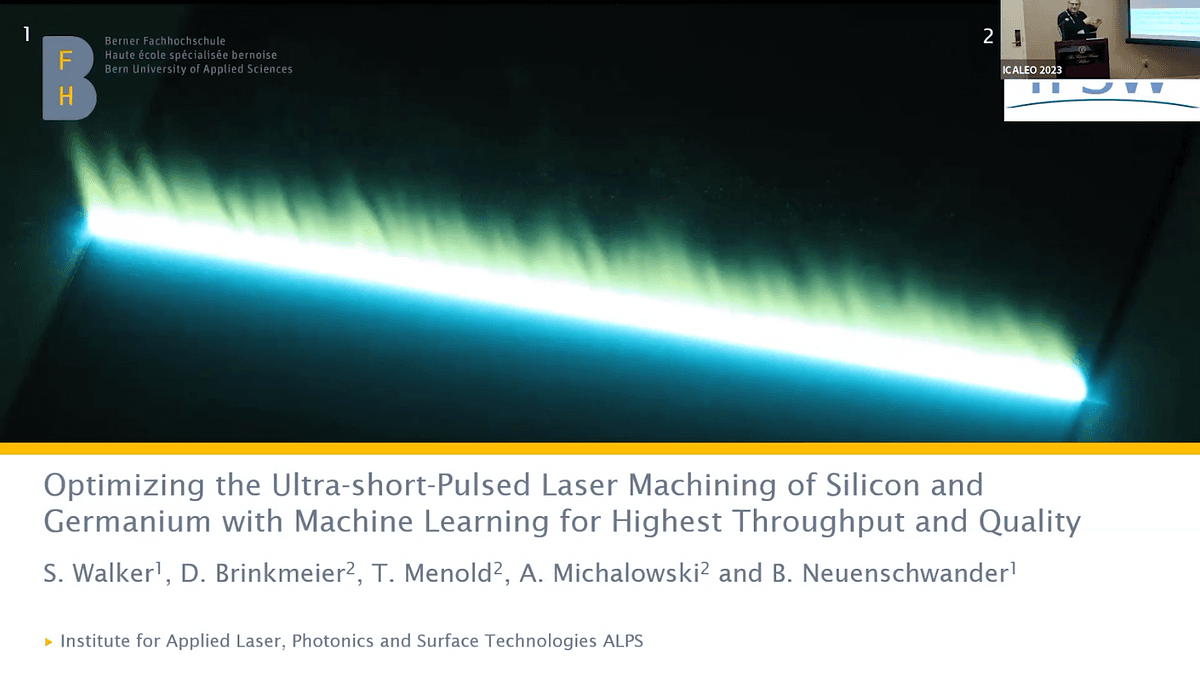
Premium content
Access to this content requires a subscription. You must be a premium user to view this content.
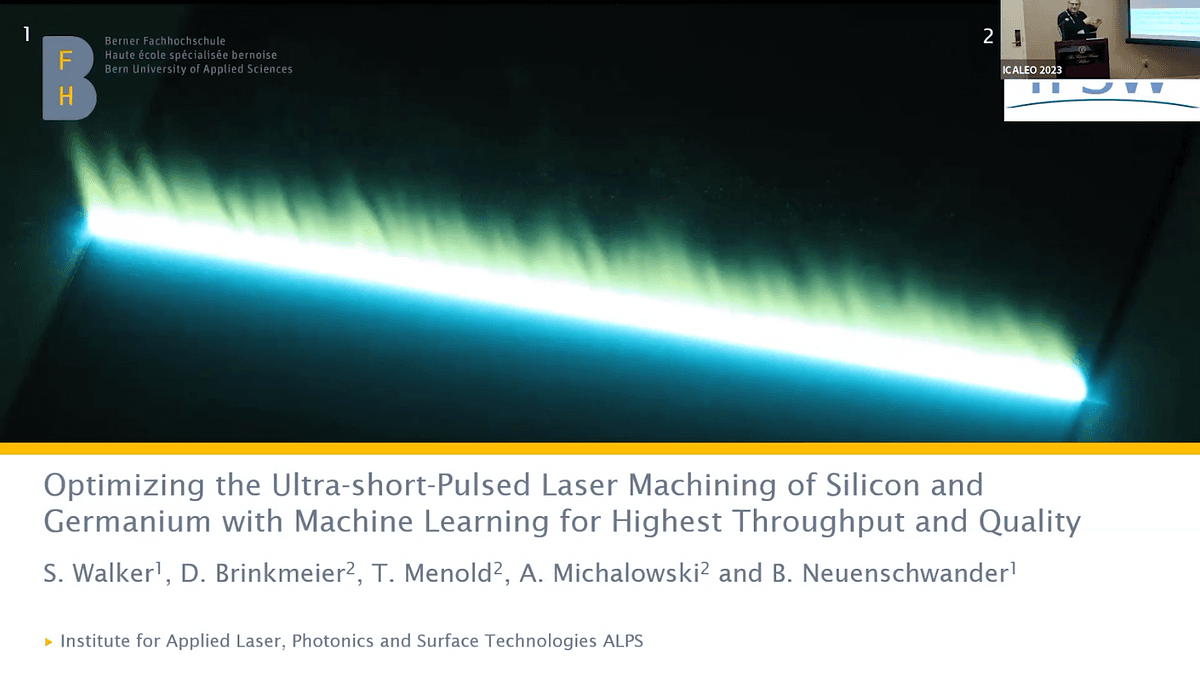
technical paper
Optimizing the Ultra-Short-Pulsed Laser Machining of Silicon and Germanium With Machine Learning for Highest Throughput and Quality
keywords:
ultra-short-laser pulses
process optimization
semiconductor
artificial intelligence
machine learning
Modern beam sources and system technology allows to adjust a large number of parameters relevant to the machining process, and often these parameters can even be varied as a function of time. In principle, a large parameter space makes it possible to find and use particularly well-suited parameter regimes for the specific application, but this generally increases the experimental effort considerably. The efficient optimization of process parameters, incorporating all the possibilities of modern flexible laser beam sources and system technology, in perspective also opens the door to high quality and high throughput.
Semiconductors as Silicon and Germanium show on the one hand significantly higher ablation efficiencies when they are machined with bursts but then suffers from lower machining quality. On the other hand, especially (100) oriented wafers, can be machined with very low surface roughness due to a melting effect ocuring if a certain peak fluence is exceeded. Combination of these two processes would further exceed the parameter space for a process optimization when high throughput and surface quality is demanded. We will demonstrate the potential of machine learning to significantly shorten the demanded time and effort for the process optimization when these materials are machined with ultra-short laser pulses.