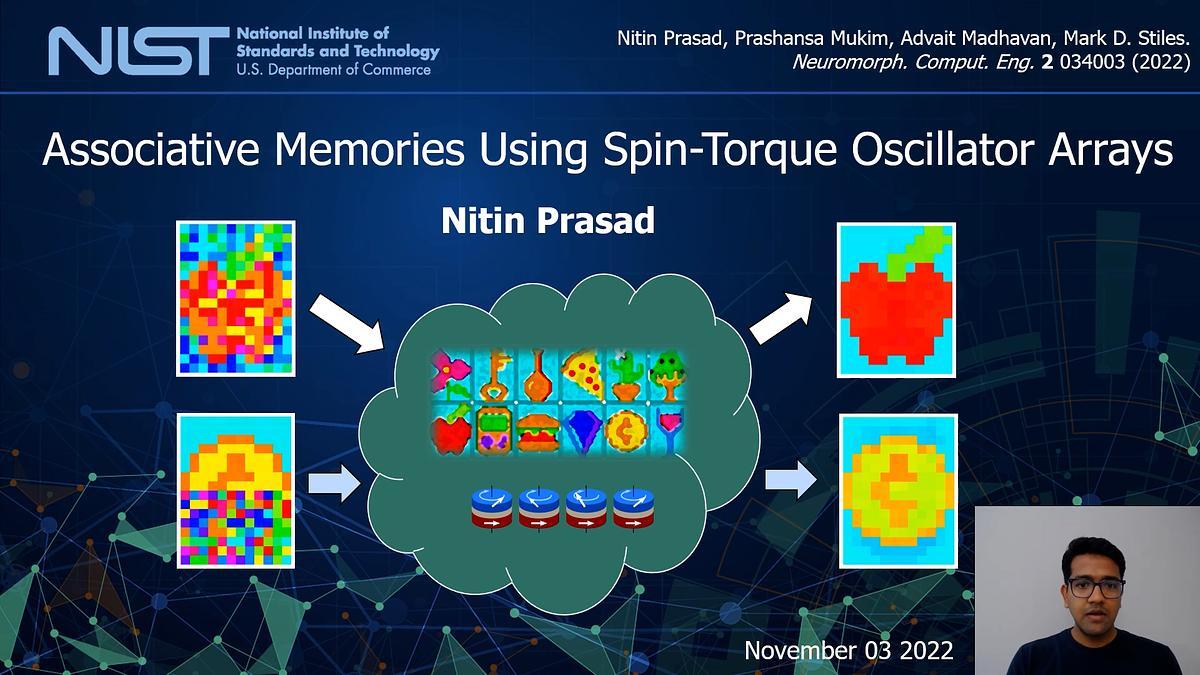
Premium content
Access to this content requires a subscription. You must be a premium user to view this content.
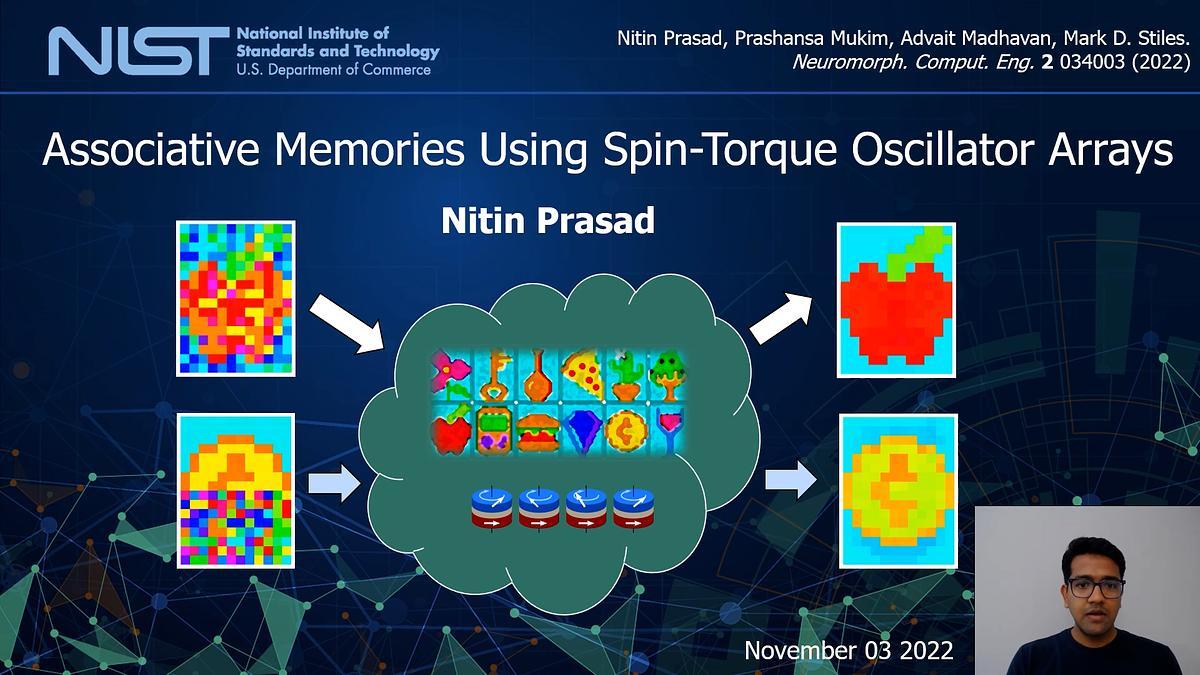
technical paper
Associative Memories using Spin Torque Oscillator Arrays
The parallels between associative learning observed in the brain and spontaneous synchronization observed in weakly coupled oscillators have led to proposals for artificial associative memories based on synchronized oscillators 1,2. Here, we consider spin-torque oscillator arrays to form a frequency synchronized oscillator network 1. Spin-torque oscillators are capable of frequency locking to external AC drives, provided the external drive frequencies are close to their natural frequencies 3. Information is encoded in the coupling network while the relative phases between the frequency-synchronized oscillators encode the current state of the network. There are several possible topologies to implement the coupling networks 2 and the couplings themselves can be implemented either using magnetostatic and exchange interactions 3, or more easily though electrical circuitry.
Here, we consider an all-to-all pairwise-connected feedback network, as shown in Fig. 1 with each connection representing a complex weight 4. These complex weights consist of time-delay and scaling networks implemented using memristor-augmented CMOS circuitry. When connected in feedback, the relative phases of the oscillators stabilize into one of the states corresponding an encoded vector in the feedback network. We demonstrate storage and retrieval of twelve 16×12 images using 192 spin-torque oscillators. The pixel values of the images considered are drawn from a saturated color wheel which naturally maps to the phase of the oscillator corresponding to that pixel. A schematic of the phase evolution during a typical retrieval process is shown in Fig. 2. Starting from a corrupt state, the oscillator phases evolve in the presence of feedback so that the final image corresponds to one of the stored images that closely resembles the corrupt image. For the oscillators and circuitry considered, the image retrieval process when presented with an image with 5 % root mean square deviations from the ideal image requires approximately 5 μs and consumes roughly 130 nJ. These simulations show that for the network to function as desired, the resonant frequencies of the oscillators are required to have fractional spread lower 0.01 %.
References:
1 N. Prasad, P. Mukim, A. Madhavan, and M. D. Stiles. arXiv preprint arXiv:2112.03358 (2022).
2 D. E. Nikonov, G. Csaba, W. Porod, et al. IEEE J. Explor. Solid-State Comput. Devices Circuits 1, 85 (2015).
3 S. Kaka, M. R. Pufall, W. H. Rippard, et al. Nature 437, 389 (2005).
4 S. Jankowski, A. Lozowski, and J. M. Zurada. IEEE Trans. Neural Netw. 7, 6 (1996).