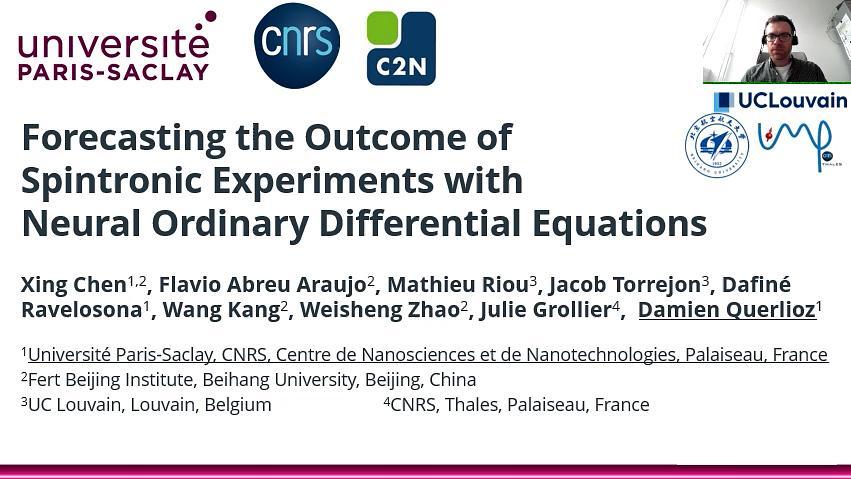
Premium content
Access to this content requires a subscription. You must be a premium user to view this content.
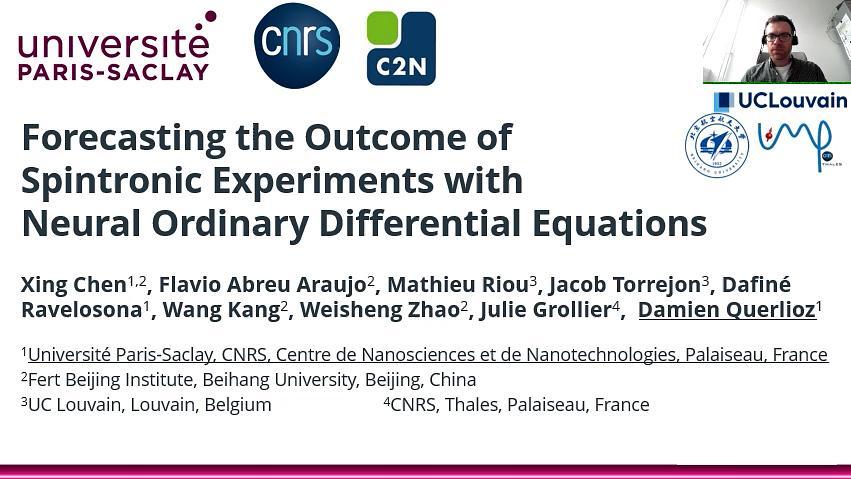
technical paper
Forecasting the Outcome of Spintronic Experiments with Neural Ordinary Differential Equations
Reaching the full potential of spintronics requires modeling to predict the results of spintronic experiments, optimize structures, and design systems. Currently, the gold standard for modeling spintronic structures is micromagnetic simulations. Unfortunately, these simulations can be extremely slow and struggle with modeling accurately the imperfections
and noise inherent to experimental devices. In this work, we introduce an alternative approach: design models for spintronic devices by letting an artificial intelligence automatically
generate a model based on examples of the device behavior. For this, we make use of the recently-proposed formalism of Neural Ordinary Differential Equations (Neural ODE) 1, which
we adapt to the specificities of spintronics 2. An appropriately-trained Neural ODE can simulate a skyrmion-based device 200 times faster than micromagnetic simulations while being
highly accurate, even in complex situations and for multiple values of materials parameters (magnetic anisotropy, DMI). We also train a Neural ODE based on five milliseconds of
experimental measurements of vortex-based spin-transfer nano oscillators. The Neural ODE could then predict weeks of experimental measurements. We show how to adapt the Neural
ODE, an originally deterministic formalism, to incorporate noise and show that the predictions of the Neural ODE match the experiments very precisely. All these results highlight the
potential of Neural Ordinary Differential Equations for developing spintronic applications.
References
1 R. T. Chen, Y. Rubanova, J. Bettencourt, and D. K. Duvenaud, "Neural Ordinary Differential Equations", Advances in Neural Information Processing Systems, 6571–6583
(2018).
2 X. Chen, F. A. Araujo, M. Riou, J. Torrejon, D. Ravelosona, W. Kang, W. Zhao, J. Grollier, D. Querlioz, "Forecasting the outcome of spintronic experiments with Neural Ordinary
Differential Equations", Nature Communications 13, 1016 (2022).