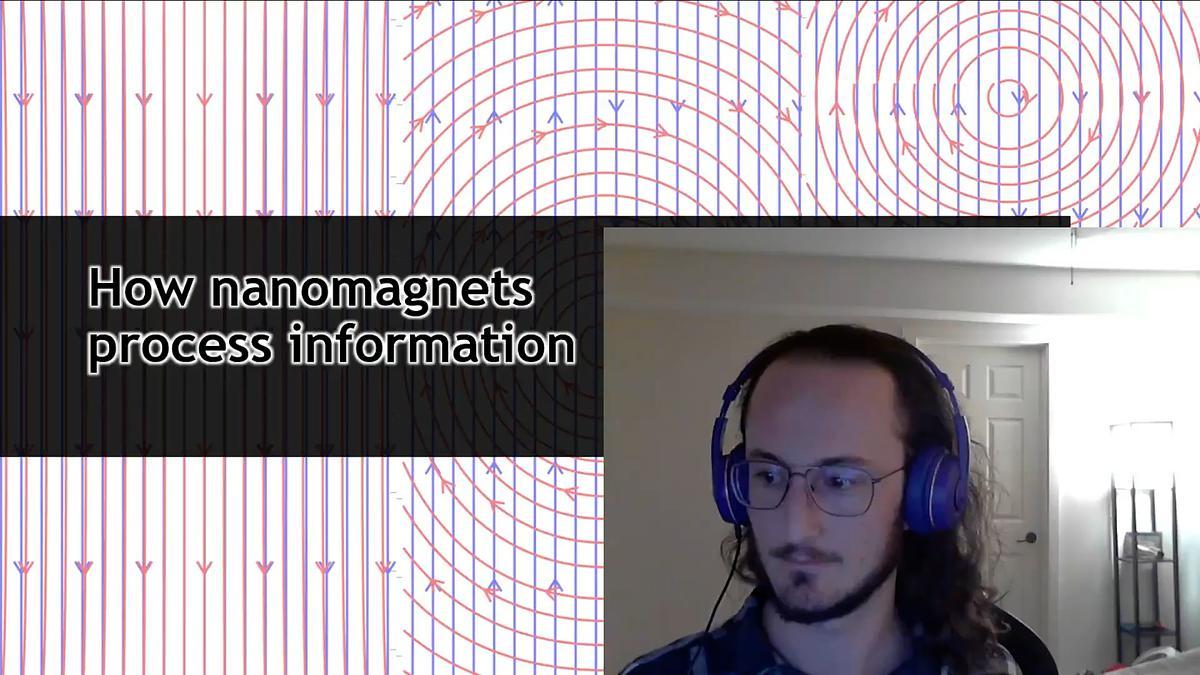
Premium content
Access to this content requires a subscription. You must be a premium user to view this content.
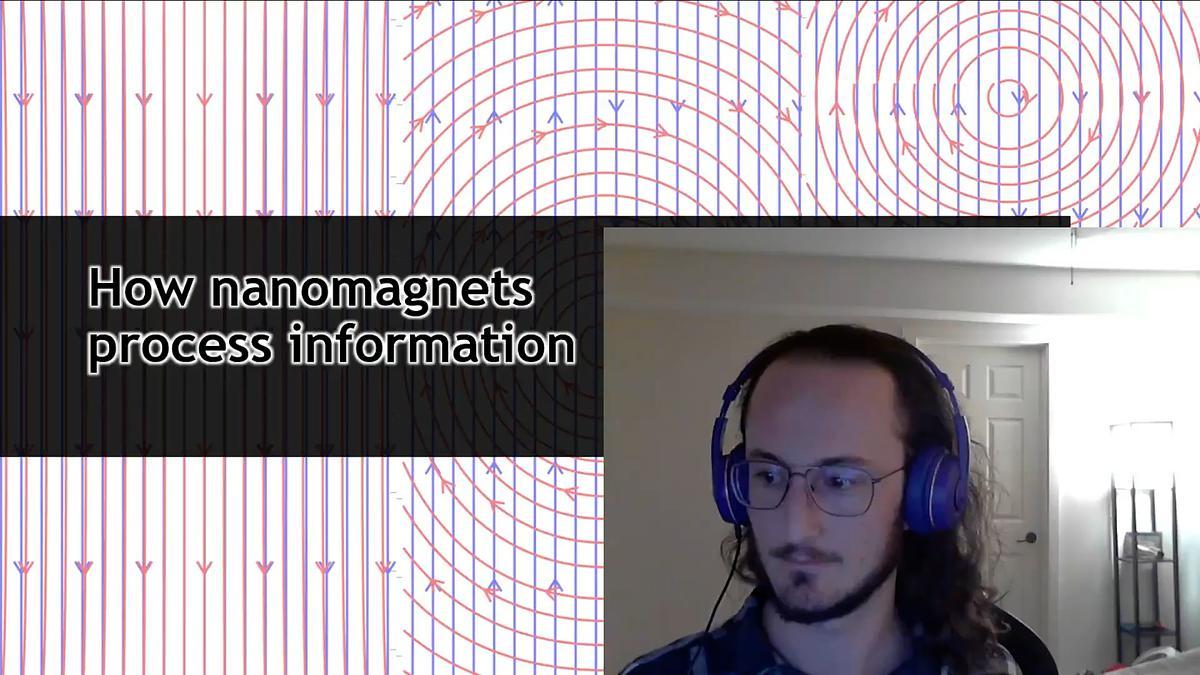
technical paper
How nanomagnets process information
As the era of Moore's law in transistor based computing comes to an end, it is possible that society will rely on new systems to process information 1. Nanomagnets are an appealing candidate to supercede transistors as they may theoretically reach the Landauer limit of efficiency at room temperature 2. Arrays of magnetic material may be patterned in nearly any 2D pattern conceivable and made of a myriad of materials to engineer a wide variety of phases, from standard ferromagnetic order to skyrmions. The interactions between domains creates Ising like behavior which naturally maps onto a variety of computing schemes, such as nanomagnetic logic 3, Hopfield networks 4, and QUBO algorithms 5. The nonlinear field response even effectively performs the function of a reservoir in the neuromorphic computing framework of "reservoir computing" 6. This richness of behaviors is messy and more difficult to control than CMOS systems, but offers incredible efficiency and naturally organic structure if given the appropriate problems. Artificial spin ice, nanomagnet arrays patterned to simulate the difficult to study problem of frustrated magnetism 7, has recently branched into the field of nanomagnetic computing. Here we will incorporate principles from artificial spin ice to better understand how interacting nanomagnets process information. We will also introduce a model for the mean-field dynamics of nanomagnets that illuminates how external field can drive collective behavior in such a way that the spin system's dynamics perform useful information processing. We then apply the results of this model to reservoir computing to attain a lower error signal prediction framework comprised of nanomagnets.
References:
1 T. Theis, and H-S. P. Wong, Computing in Science & Engineering., Vol. 19.2, p.41-50 (2017)
2 B. Lambson, D. Carlton, and J. Bokor, Physical review letters., Vol. 107.1, p.010604 (2011)
3 H. Arava, et al, Nanotechnology, Vol. 29.26, p.265205 (2018)
4 M. Saccone, et al, Nature Physics, Vol. 18.5, p.517-521 (2022)
5 A. Lucas, Frontiers in physics, Vol. 1 p.5 (2014)
6 J. Gartside, et al, Nature Nanotechnology, Vol. 17.5, p.460-469 (2022)
7 S. Skjærvø, et al, Nature Reviews Physics, Vol. 2.1, p.13-28 (2020)