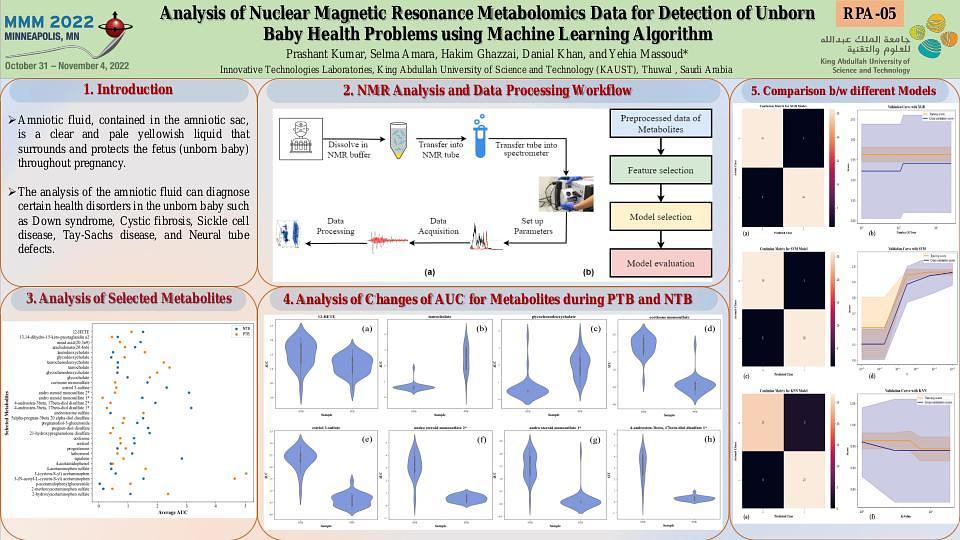
Premium content
Access to this content requires a subscription. You must be a premium user to view this content.
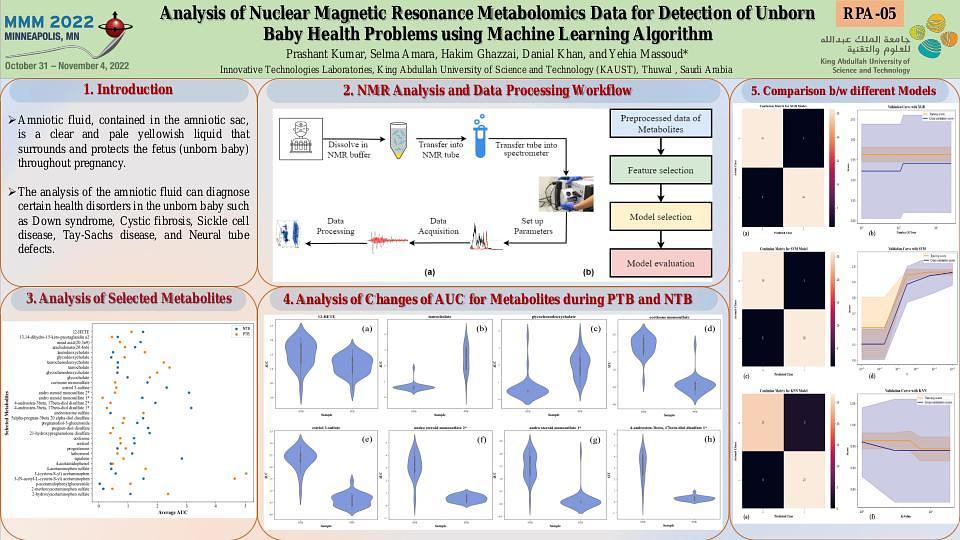
poster
Analysis of Nuclear Magnetic Resonance Metabolomics data for the detection of unborn baby health problems using deep learning
Amniotic fluid is a clear and pale yellowish liquid that surrounds and protects the fetus (unborn baby) throughout pregnancy 1. The amniotic fluid analysis can diagnose certain health disorders in the unborn baby such as Down syndrome, Cystic fibrosis, Sickle cell disease, Tay-Sachs disease, and Neural tube defects 2. Fig. 1 shows a 10-week-old human fetus surrounded by amniotic fluid within the amniotic sac 3.
This abstract presents a deep learning/machine learning pipeline that can be used for the prediction of health problems in an unborn baby by using deep learning or machine learning algorithm. The work is divided into two parts; (1) data collection, and (2) data processing and analysis. Fig. 2(a) shows data collection workflow. In the data collection, 20 samples of amniotic fluid are collected from a healthy and an unhealthy woman during 15~20 weeks of pregnancy 4. After collecting the samples, the nuclear magnetic resonance (NMR) is performed for data acquisition 5. Fig. 2(b) depicts workflow of data processing and analysis. In this part, metabolites data is normalized and a data reduction method like PCA is applied. After getting the preprocessed data, feature selection algorithms are performed to remove the irrelevant data. Three variable selection algorithms such as (i) Correlation-based feature selection (CFS), (ii) Partial least squares regression (PLS), and (iii) Learning Vector Quantization (LVQ) 4 are used. For each selected set of metabolites, support vector machine (SVM) models are generated using 10-fold cross-validation. To evaluate the performance and significance of the optimized model, we perform a permutation test. In the 10-fold cross-validation, the permutation test shuffles the class labels while using the same set of metabolites. For data analysis, we calculate true/false positives and true/false negatives for each round of our 10-fold cross-validation to report average sensitivity (TP/P) and specificity (TN/N) values along with the average of the area under the curve (AUC) 4.
References:
1. C. M. Broek, J. Bots, I. V. Lasheras, M. Bugiani, F. Galis, S. V. Dongen, “Amniotic fluid deficiency and congenital abnormalities both influence fluctuating asymmetry in developing limbs of human deceased fetuses,” PLoS One, vol. 8, no. 11, 2013.
2. MedlinePlus Internet, National Library of Medicine (US), “Amniocentesis (amniotic fluid test),” Available from: https://medlineplus.gov/lab-tests/amniocentesis-amniotic-fluid-test/.
3. https://en.wikipedia.org/wiki/Amniotic_fluid.
4. R. O. B. Singh, A. Yilmaz, H. Bisgin, O. Turkoglu, P. Kumar, E. Sherman, A. Mrazik, A. Odibo, and S. F. Graham, “Artificial intelligence and the analysis of multi-platform metabolomics data for the detection of intrauterine growth restriction,” PLoS One vol. 14, no. 4, 2019.
5. https://www.jeol.co.jp/en/products/nmr/basics.html.