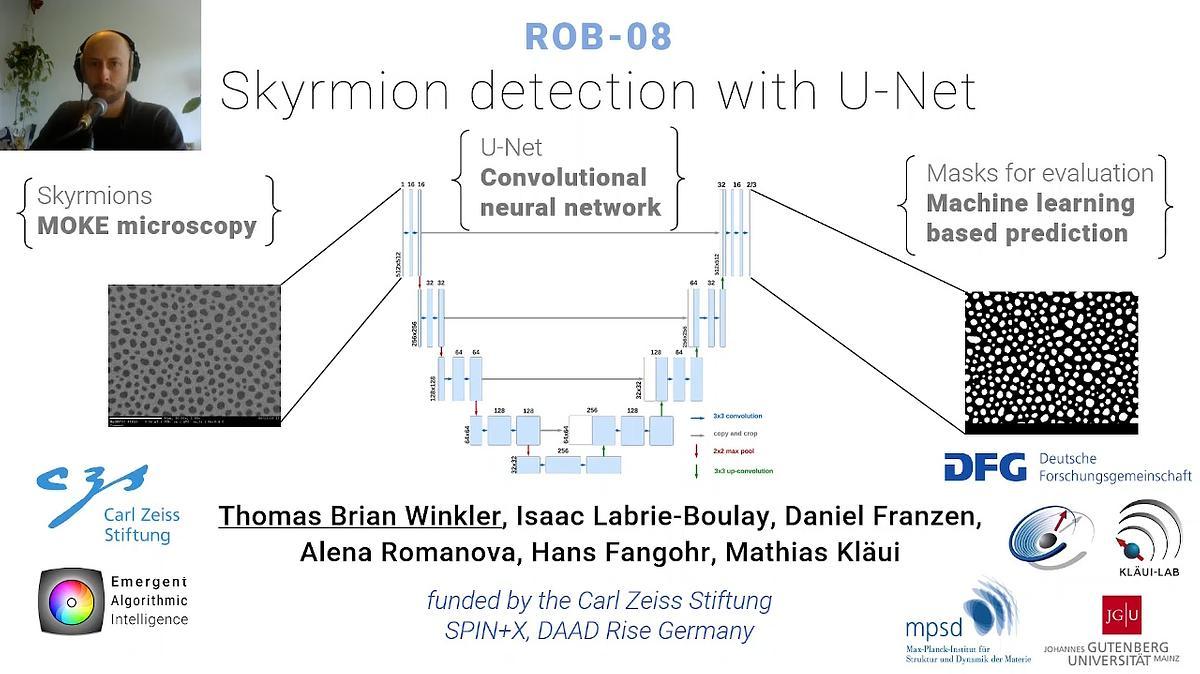
Premium content
Access to this content requires a subscription. You must be a premium user to view this content.
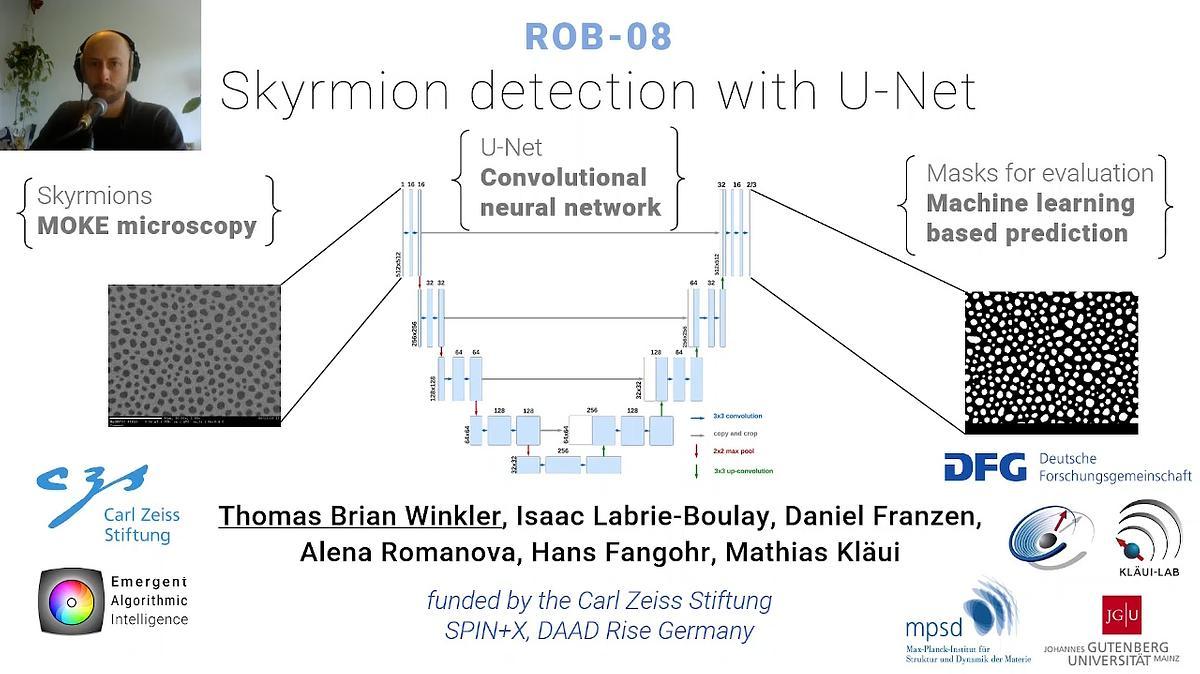
technical paper
Skyrmion detection with U Net
Magnetic skyrmions 1 are topologically stabilized, magnetic quasi-particles, which exhibit interesting physical phenomena and are also potential candidates for efficient neuromorphic computation schemes. On the micro-meter scale, they are detectable with Kerr-microscopy, using the magneto-optical Kerr-effect. However, depending on the material stack, temperature, the growth procedure and other influences, those measurement might suffer from noise, low-contrast, intensity gradients or defects, therefore manual data treatment is normally necessary to generate data that can be evaluated easily.
Our approach is to use a convolutional neural network, based on the U-Net 2, to detect the position and shape of the skyrmions in our measurement data. This work was motivated by reports that machine learning has been successfully applied to (micro-)magnetic problems 3. We are tuning the U-Net with various techniques to optimize the predictions and identify skyrmions and defects fast and with high reliability. A well-trained neural network is shown to minimize manual treatment of data. The approach is also easily extendable to other magnetic structures.
References:
1 Everschor-Sitte et al., Journal of Applied Physics 124, 240901 (2018)
2 Ronneberger et al., MICCAI 2015. Lecture Notes in Computer Science, vol 9351. Springer, Cham. (2015)
3 Alexander Kovacs et al., Journal of Magnetism and Magnetic Materials 491, 165548 (2019)