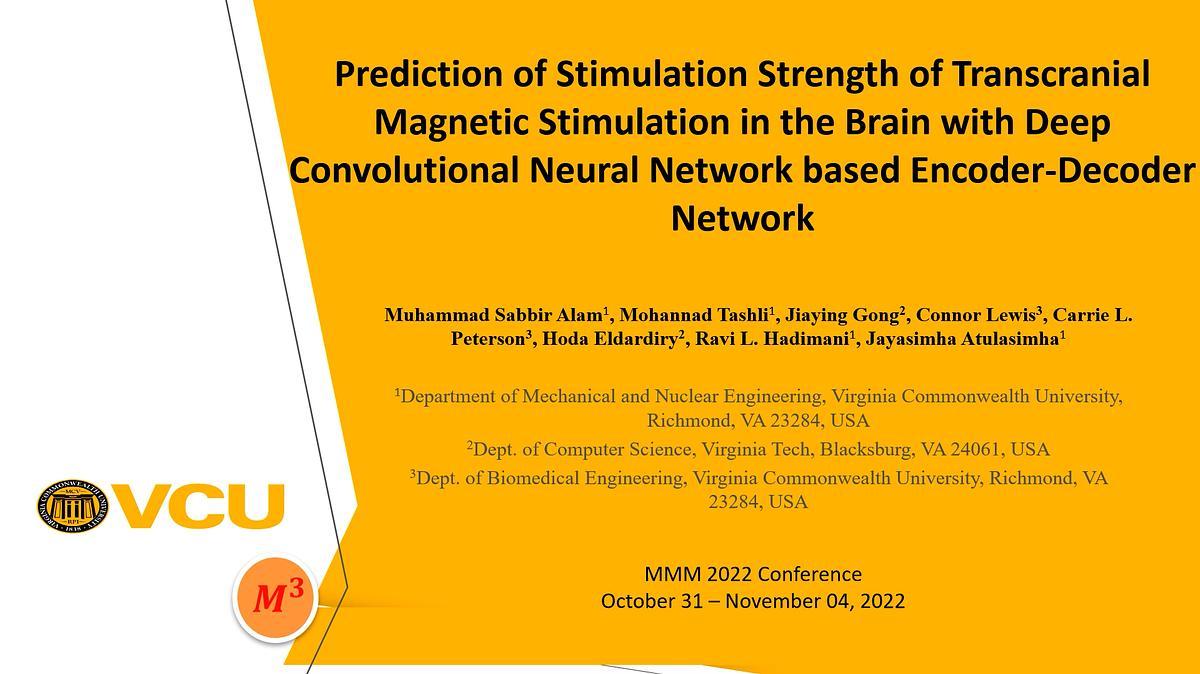
Premium content
Access to this content requires a subscription. You must be a premium user to view this content.
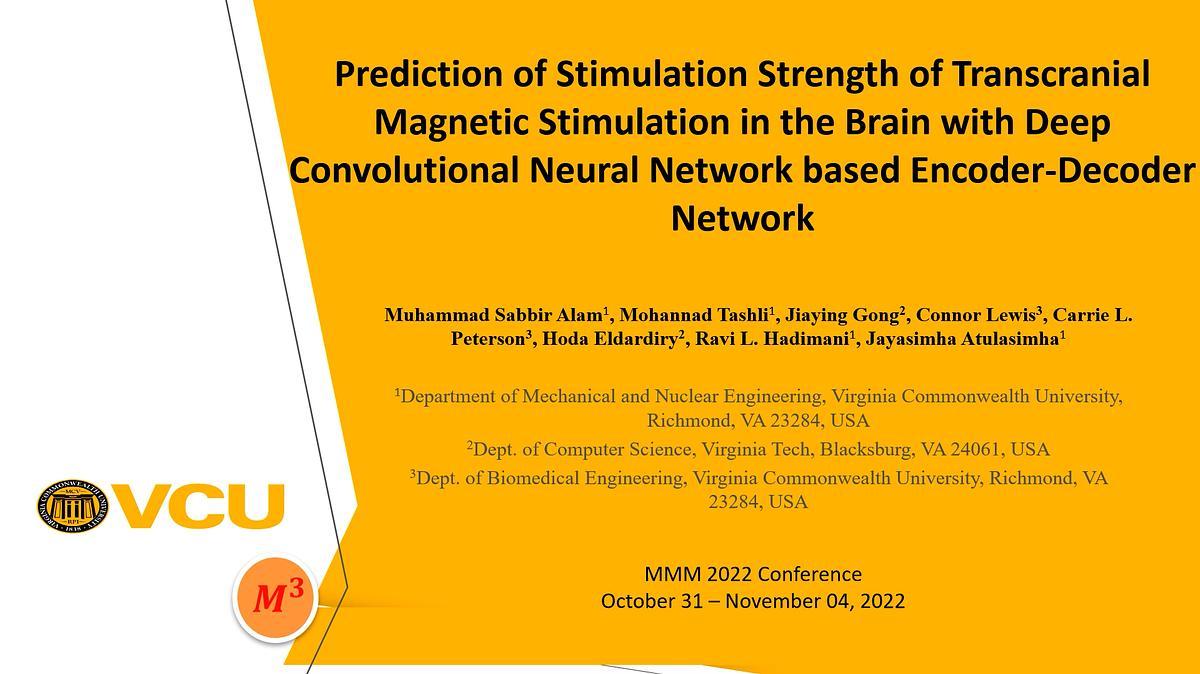
technical paper
Prediction of Stimulation Strength of Transcranial Magnetic Stimulation in the Brain with Deep Convolutional Neural Network based Encoder Decoder Network
Transcranial magnetic stimulation (TMS) is a non-invasive, effective, and safe neuromodulation technique used to diagnose and treat neurological disorders 1-2. A key
challenge involved with TMS is to determine stimulation strength in critical regions of the brain accurately. The induced electric field is affected by complexity and heterogeneity of the
brain and numerical computation methods like finite element analysis (FEA) can be used to estimate electric field distribution. However, these methods need exceedingly high
computational resources and are time-consuming 3-6. The purpose of this study was to develop and test a machine learning algorithm that can efficiently predict stimulation strength
based on neuroanatomy. Head models were developed from magnetic resonance images of eleven recruited subjects using SimNIBS pipeline 7-8. We used Sim4Life, an FEA software
to compute induced electric fields due to simulated TMS; the induced electric fields served as training data. A deep convolutional neural network (DCNN) inspired encoder-decoder
network (Fig 1) was developed to predict induced electric fields from T1-weighted and T2-weighted magnetic resonance imaging (MRI) based anatomical slices. Three representative cases
of electric fields predicted by the DCNN algorithm, along with the corresponding reference electric fields are shown in Fig 2. For the trained network, the training data and the testing data
peak signal to noise ratios are 32.83dB and 28.01dB respectively. The key contribution of this study is the ability to predict the induced electric fields in real-time as well as accurately and
efficiently predicting TMS strength in the targeted brain regions saving both time and computational resources.
Acknowledgment: This work was supported by VCU CERSE. Further funding was provided by the CCI CVN (Proposal ID #: FP00010500).
References:
1 D. J. Stultz, S. Osburn, T. Burns, S. Pawlowska-Wajswol, and R. Walton, Transcranial magnetic stimulation (TMS) safety with respect to seizures: A literature review,
Neuropsychiatric disease and treatment, vol. 16, p. 2989 (2020).
2 M. Kobayashi, and A. Pascual-Leone, 2003. Transcranial magnetic stimulation in neurology, The Lancet Neurology, vol. 2, no. 3, p. 145-156 (2003).
3 E. G. Lee, W. Duffy, R. L. Hadimani, M. Waris, W. Siddiqui, F. Islam, M. Rajamani, R. Nathan, and D. C. Jiles, Investigational effect of brain-scalp distance on the efficacy of
transcranial magnetic stimulation treatment in depression, IEEE Transactions on Magnetics, vol. 52, no. 7, p. 1–4 (2016).
4 L. J. Crowther, R. L. Hadimani, A. G. Kanthasamy, and D. C. Jiles, Transcranial magnetic stimulation of mouse brain using high-resolution anatomical models, Journal of Applied
Physics, vol. 115, no. 17, p. 17B303 (2014).
5 F. Syeda, H. Magsood, E. G. Lee, A. A. El-Gendy, D. C. Jiles, R. L. Hadimani, Effect of anatomical variability in brain on transcranial magnetic stimulation treatment, AIP Advances,
vol. 7, no. 5, p. 056711 (2017).
6 F. Syeda, K. Holloway, A. A. El-Gendy, and R. L. Hadimani, Computational analysis of transcranial magnetic stimulation in the presence of deep brain stimulation probes, AIP
Advances, vol. 7, no. 5, p. 056709 (2017).
7 N. Mittal, C. Lewis, Y. Cho, C. L. Peterson, and R. L. Hadimani, Effect of fiber tracts and depolarized brain volume on resting Motor thresholds during transcranial magnetic
stimulation, IEEE Transactions on Magnetics (2022).
8 N. Mittal, B. Thakkar, C. B. Hodges, C. Lewis, Y. Cho, R. L. Hadimani, and C. L. Peterson, Effect of neuroanatomy on corticomotor excitability during and after transcranial magnetic
stimulation and intermittent theta burst stimulation, Human Brain Mapping (2022).