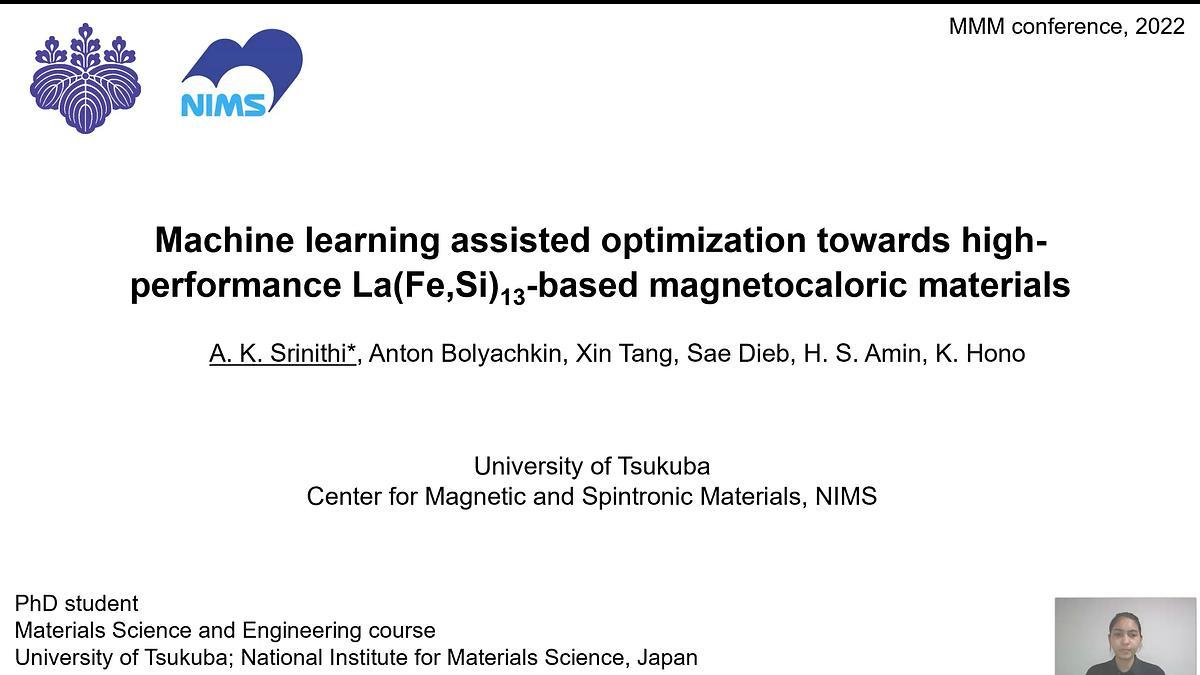
Premium content
Access to this content requires a subscription. You must be a premium user to view this content.
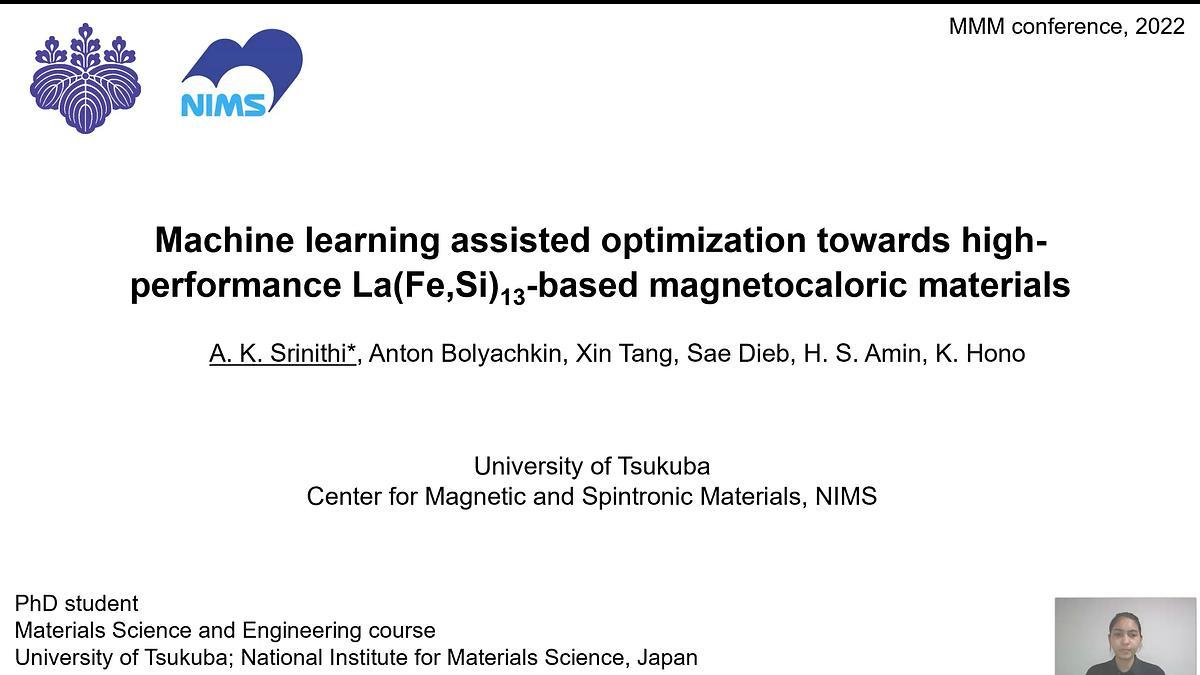
technical paper
Machine learning assisted optimization towards high performance La(Fe,Si)13
La(Fe,Si)13-based (1:13) compounds exhibit giant magnetocaloric effect and are promising candidates for room temperature as well as cryogenic magnetic refrigeration
1,2. The magnetic refrigeration materials require a comprehensive evaluation of their properties such as transition temperature (Ttr), entropy change (△Sm), adiabatic temperature change
(△Tad), thermal hysteresis (△Thys), relative cooling power (RCP), thermal conductivity and mechanical stability 3. Extensive studies on tuning these properties have been carried out for
the 1:13 system by varying the chemical compositions and processing conditions. However, an excellent combination of properties has not been achieved yet, hindering any practical
application 4. In this work, we employed machine learning (ML) to address the optimization problem in the 1:13 system. A dataset containing over 1000 samples in total was collected
using data mining across 200 articles. Several machine learning models were trained to predict Ttr, △Sm, RCP and Thys as targets and compared using the root mean squared error (RMSE)
metric. We found that random forest (RF) and gradient boosting (GB) regressors outperformed other models such as decision tree and k-NN regressors. The achieved performance of the
former model is demonstrated in Fig. 1 for the case of predicting Ttr. The relative feature importance extracted from the trained RF model in Fig. 2 shows the important parameters for
tuning the Ttr, while the corresponding Pearson correlation co-efficients (PCC) shows how these parameters influence the Ttr. Similarly, the critical parameters were found out for the other
target properties and used for further optimization study. The compositional optimization of the 1:13 system was performed by means of the differential evolution technique with a multiobjective
target toward the best combination of the magnetocaloric properties.
References:
- A. Fujita et al., Phys. Rev. B, 67 (2003) 104416.
- S. Fujieda et al., Appl. Phys. Lett., 89 (2006) 062504.
- V. Franco et al., Annual Review of Materials Research, 42 (2012) 305-342.
- V. Paul-Boncour et al., Magnetochemistry, 7 (2021) 13.