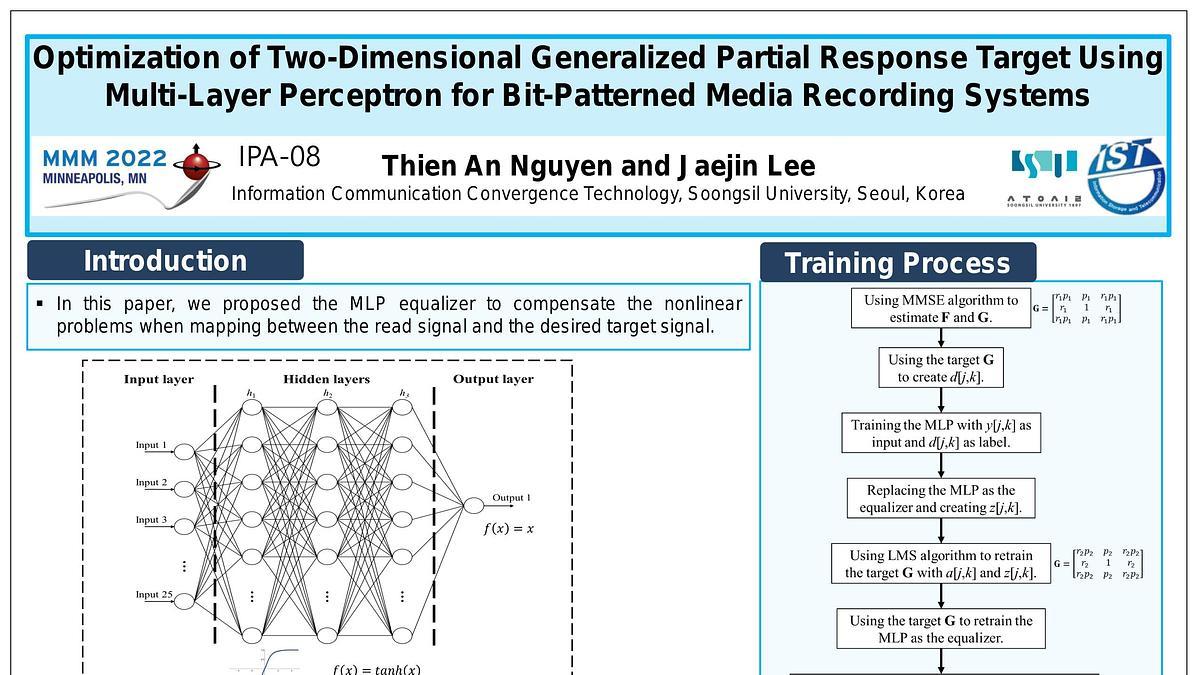
Premium content
Access to this content requires a subscription. You must be a premium user to view this content.
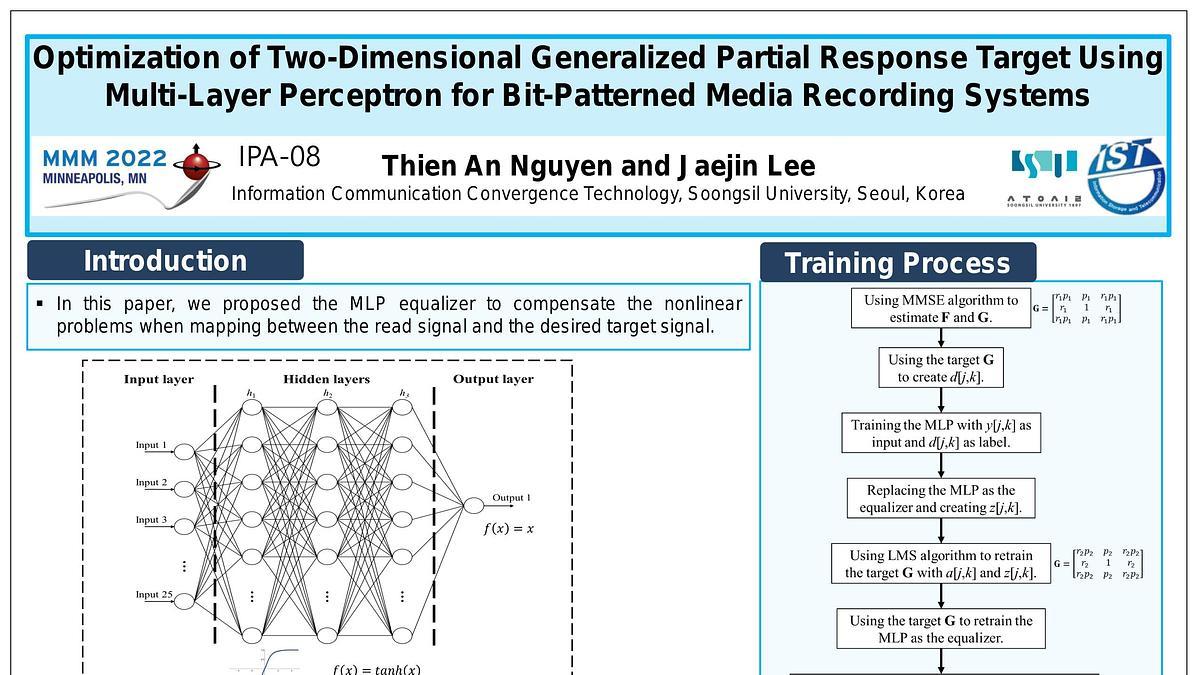
poster
Optimization of Two Dimensional Generalized Partial Response Target Using Multi
In bit patterned media recording (BPMR), the areal density is increased by reducing the distance between the magnetic islands. Thus, the BPMR faces a two-dimensional (2D) interference problem. To solve the 2D interference, the output of the BPMR channel is passed through the equalizer to convert it into the desired signal, which has the known coefficient interference. However, mapping between the output of the BPMR channel and the desired signal is a nonlinear problem. Therefore, the nonlinear equalizer based on neural network structure is proposed in 1. However, because the training process is too complicated, the equalizer based on the neural network is used to estimate the 1D generalized partial response (GPR) target. To improve the equalizer based on the neural network, we introduce the neural network equalizer and method to train the neural network equalizer with a 2D GPR target. In the proposed model (Fig. 1), firstly, we use the method in 2 to estimate the parameters of the serial target (step 0). These target coefficients are kept to create the desired signal dj,k as the label for the training process of the neural network. To train the neural network, we use the received signal yj,k as the input and the signal dj,k as the label (step 1). Next, we re-train the target’s coefficients by using the trained neural network to create the yj,k as the label and the modulated signal aj,k as the input (step 2). Finally, the neural network is re-trained using the target coefficients from step 2 and remaking step 1 (step 3). Therefore, the GPR target is optimized by the nonlinear equalizer of the multi-layer perceptron (MLP). The results from the simulations (Fig. 2) show that our proposed model improves the bit error ratio (BER) performance compared to the previous studies 1 and 2 after re-training the neural network at step 3.
References:
1 J. Shen and N. Nangare, “Nonlinear Equalization for TDMR Channels Using Neural Networks,” 2020 54th Annual Conference on Information Sciences and Systems (CISS), 2020, pp. 1-6.
2 T. A. Nguyen and J. Lee, “Effective Generalized Partial Response Target and Serial Detector for Two-Dimensional Bit-Patterned Media Recording Channel Including Track Mis-Registration,” Appl. Sci., 2020.
Fig.1. Diagram of the proposed model.
Fig. 2. BER performance of the proposed model.