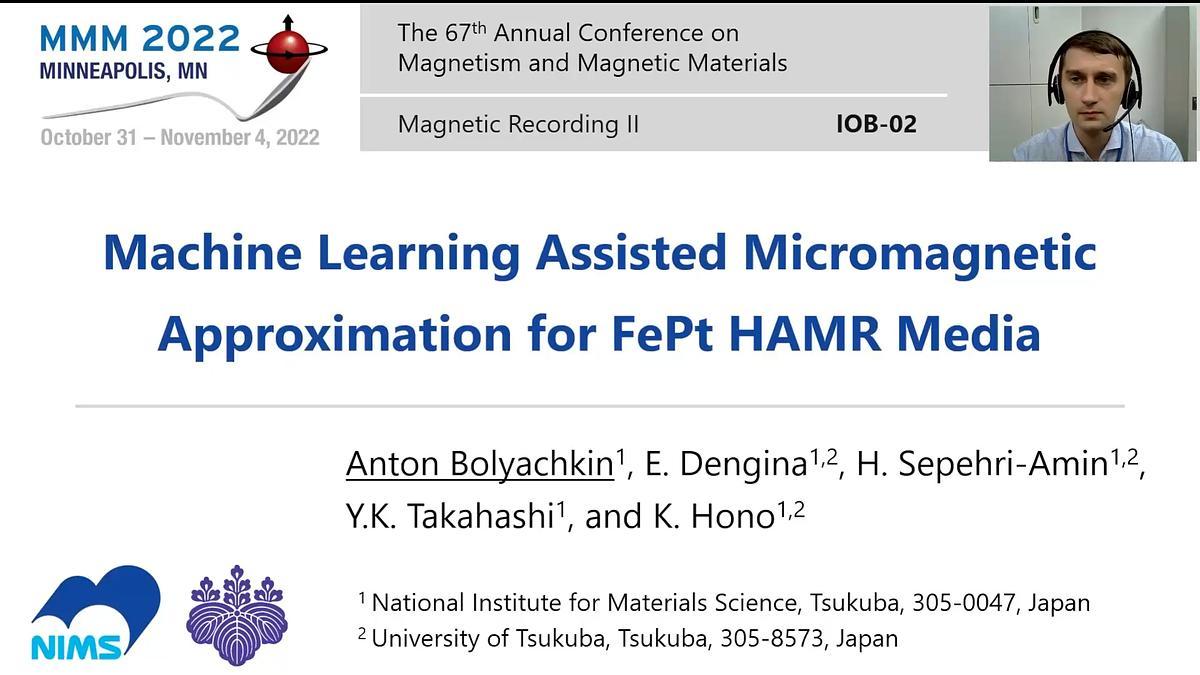
Premium content
Access to this content requires a subscription. You must be a premium user to view this content.
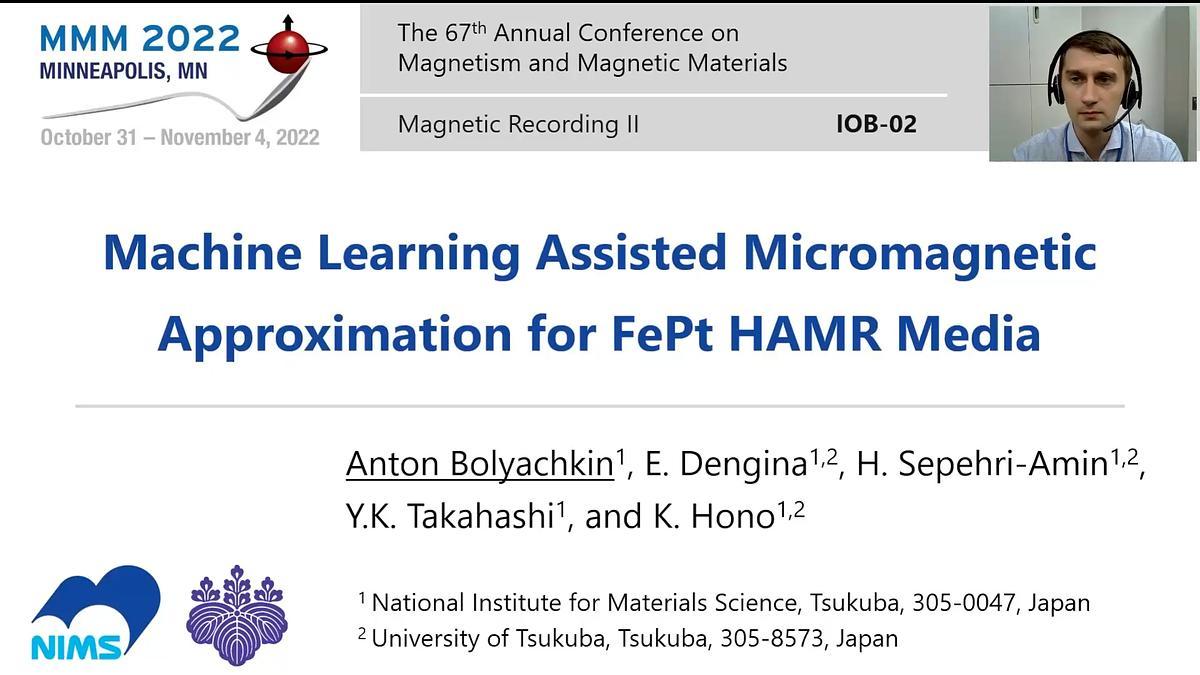
technical paper
Machine learning assisted micromagnetic approximation for FePt HAMR media
The areal recording density of hard disk drives has a potential to reach 4 Tb/in2 with the heat-assisted magnetic recording (HAMR) technology 1. So far, the best candidate for HAMR media is FePt nanogranular thin films with the L10 ordered structure. To improve the recording density and reduce the jitter noise, the bimodal grain size distribution and nanostructural defects, that are unavoidable in actual FePt films, must be suppressed. Grains with {111} twinning variants, 200 misoriented grains, and disordered grains with negligible magnetic anisotropy constants (K = 0) can be listed among the detrimental defects. Evaluation of their volume fractions, V111, V200 and VK=0 respectively, is of a vital interest.
A new micromagnetic characterization of FePt nanogranular media is proposed in this work 2. We developed a method of constructing finite element models of FePt granular media based on high-resolution transmission electron microscopy (TEM) images taken from top and side views of the films (Fig. 1). Using the TEM image based model, an accurate micromagnetic approximation of the out-of-plane hysteresis loops was achieved for MgO(6 nm)/FePt-BN(1 nm)/FePt-(C,SiO2)(7 nm) thin films as shown in Fig. 2. It yielded parameters of uniaxial magnetic anisotropy (mean magnetic anisotropy constant K, its standard deviation, distortion of easy magnetization axes) and the volume fractions of the defects. Evaluated V111 and V200 were in excellent agreement with those determined by synchrotron XRD. After such an experimental validation, we collected a dataset of demagnetization curves by micromagnetic simulations upon different parameters of magnetic anisotropy and volume fractions of defects, then a neural network was trained to predict these parameters and use them as an initial set for the micromagnetic approximation that speeded up the approach significantly 3.
Thus, the developed TEM image based micromagnetic simulations assisted by machine learning can boost optimization of FePt HAMR media linking its nanostructural features to magnetic properties.
References
1 K. Hono et al., MRS Bull. 43 (2018) 93.
2 A. Bolyachkin et al., Acta Mater. 227 (2022) 117744.
3 E. Dengina et al., Scripta Mater. 218 (2022) 114797.
Fig. 1. Finite element model of FePt granular media constructed based on TEM images.
Fig. 2. Micromagnetic approximation of an experimental out-of-plane hysteresis loop.