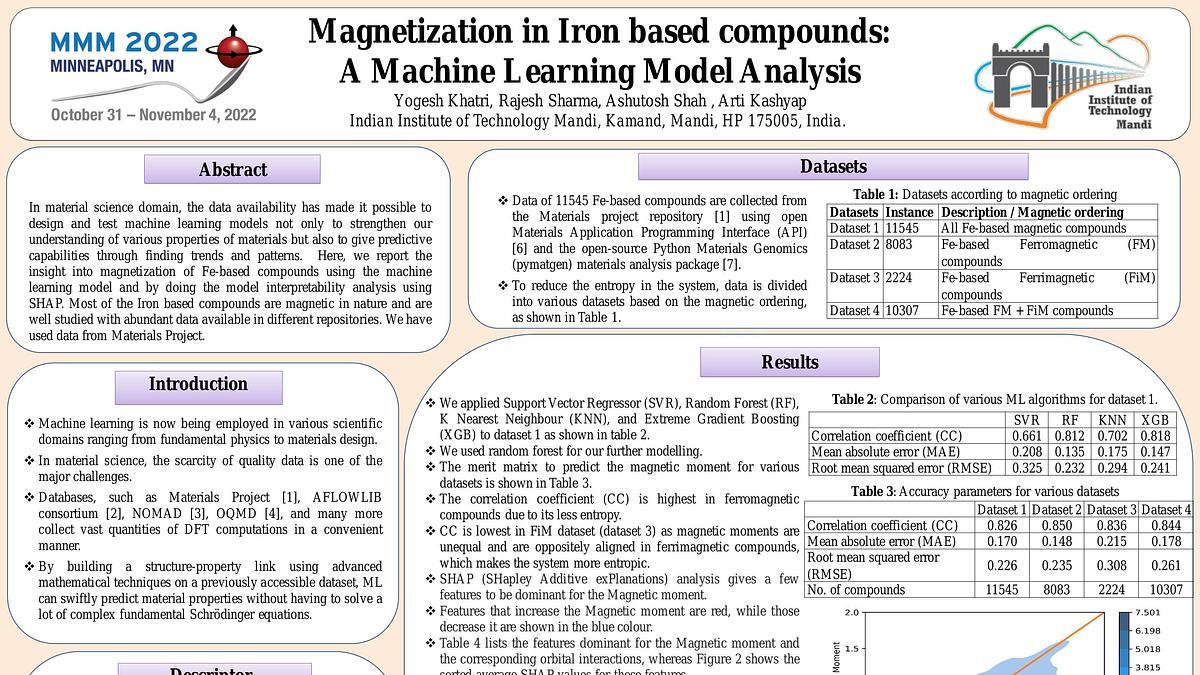
Premium content
Access to this content requires a subscription. You must be a premium user to view this content.
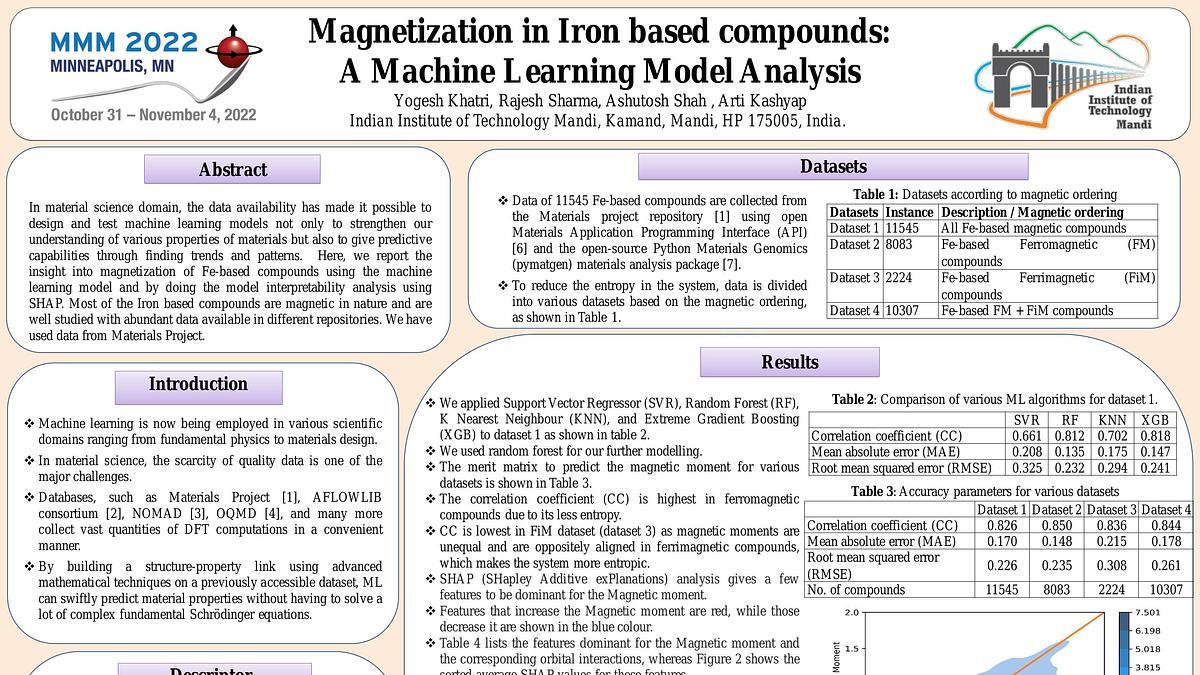
poster
Magnetization in Iron based compounds: A Machine Learning Model Analysis
The availability of large databases like materials project 1, Aflow consortium 2, NOMAD 3, OQMD 4 etc., has made it possible to apply machine learning (ML) in the materials science domain to get good predictions for various materials properties like Curie temperature 5, formation energy 6, etc. Here, we have used Materials project database to gather a data of 11545 iron-based compounds using the MAPI 7 and the pymatgen package 8. This data consists of total magnetization, crystal structure and formation energy etc for all the compounds. As a first step, we transformed the data for ML modeling by using the orbital-field matrix (OFM) representation by Pham et al. 6. In OFM, the structure of the compounds is turned into a 32*32 matrix corresponding to 1024 features, known as descriptor. With this data, we used various ML algorithms and chose to work with random forest as it provides the best results for our dataset with a 5-fold cross-validation. In order to understand the model, we used SHAP (SHapley Additive exPlanations) analysis, which is a game-theoretic approach to explain the output of any machine learning model. It gives us the most dominating features in the model. Based on the SHAP values for various datasets, the features which are dominating the overall magnetization results along with the corresponding description of the features as per the OFM descriptor are presented in Fig. 1. For example, in some oxides listed in Fig. 2, one can see that only features 173, 421, 429 are dominant as per the SHAP values. However, this is not a universally true picture for the whole dataset. Therefore, we further need to process the dataset based on the fact that not all features contribute equally in all types of materials. It is concluded that the simple ML techniques used on large dataset may give misleading results if the ML model is not improved after understanding the data we are dealing in the material science domain.
References
1 A. Jain, P. Ong, G. Hautier, S.P. Ong, W. Chen, W.D. Richards, S. Dacek, S. Cholia, D. Gunter, D. Skinner, G. Ceder, K.A. Persson, Cite as APL Mater 1 (2013) 11002.
2 S. Curtarolo, W. Setyawan, S. Wang, J. Xue, K. Yang, R.H. Taylor, L.J. Nelson, G.L.W. Hart, S. Sanvito, M. Buongiorno-Nardelli, N. Mingo, O. Levy, Comput. Mater. Sci. 58 (2012)
227–235.
3 C. Draxl, M. Scheffler, J. Phys. Mater 2 (2019) 36001.
4 J.E. Saal, S. Kirklin, M. Aykol, B. Meredig, C. Wolverton, JOM 65 (2013) 1501.
5 T. Long, N.M. Fortunato, Y. Zhang, O. Gutfleisch, H. Zhang, Mater. Res. Lett. 9:4 (2021) 169–174.
6 T. Lam Pham et.al. Sci. Technol. Adv. Mater. 18 (2017) 756–765.
7 S.P. Ong, S. Cholia, A. Jain, M. Brafman, D. Gunter, G. Ceder, K.A. Persson, Comput. Mater. Sci. 97 (2015) 209–215.
8 S.P. Ong, W.D. Richards, A. Jain, G. Hautier, M. Kocher, S. Cholia, D. Gunter, V.L. Chevrier, K.A. Persson, G. Ceder, Comput. Mater. Sci. 68 (2013) 314–319.
Fig. 1- Dominating features and corresponding orbital interaction.
Fig. 2- Few compounds with magnetization values and valence band configuration.