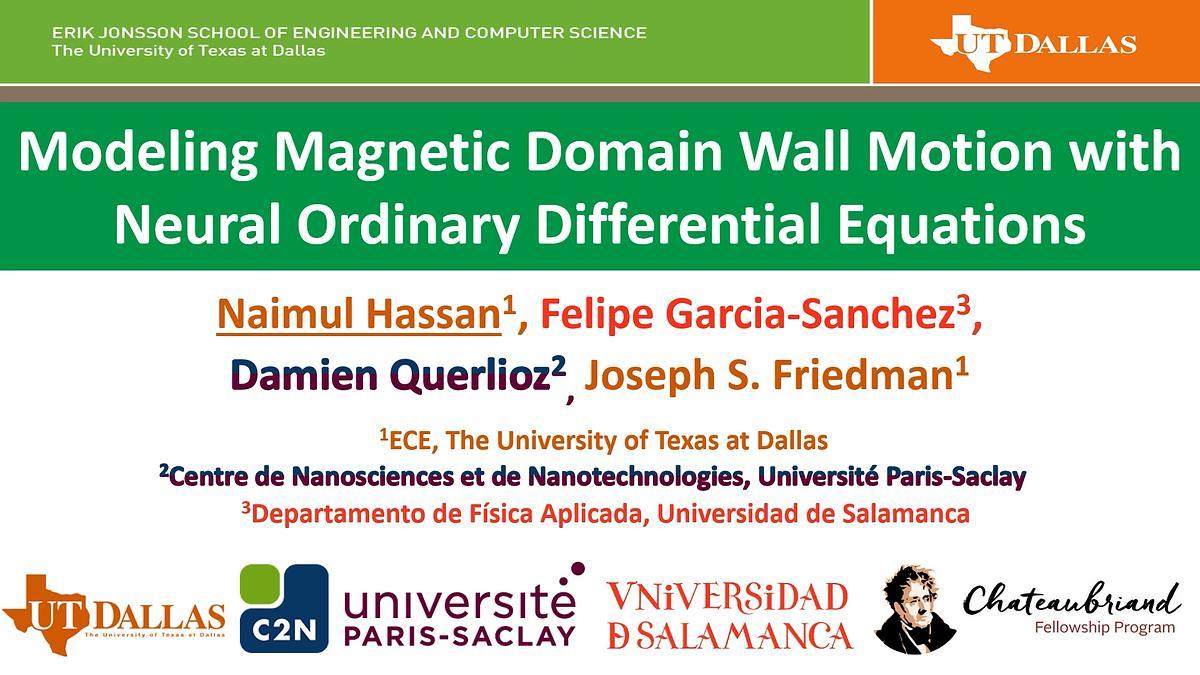
Premium content
Access to this content requires a subscription. You must be a premium user to view this content.
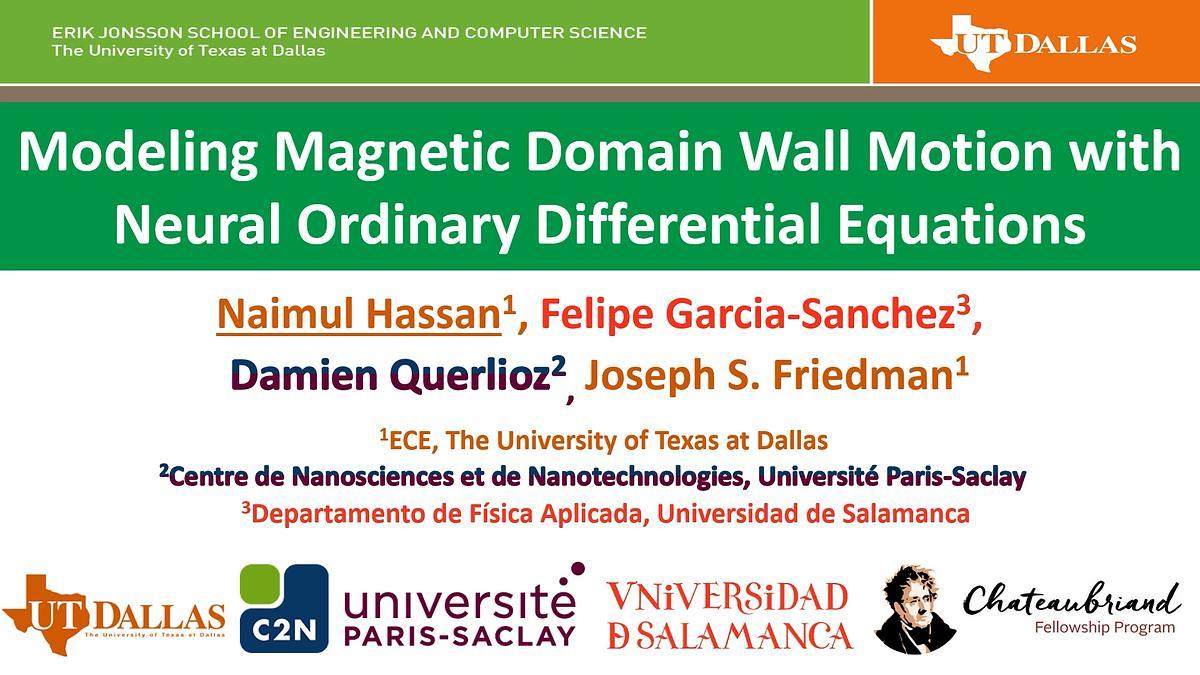
technical paper
Modeling Magnetic Domain Wall Motion with Neural Ordinary Differential Equations
The domain wall (DW) magnetic tunnel junction (MTJ) is a promising component of next-generation neuromorphic, in-memory, and logical computing hardware due to features including non-volatility, multiple analog resistive states, low power, and ultrafast read-write operation 1-3. Predicting the DW motion along a magnetic track involves complicated and time-consuming micromagnetic simulations, which greatly impede the development of computing systems designed with interconnected DW-MTJ devices 4.
The neural ordinary differential equations (ODE) is a recently conceptualized deep learning framework that can predict the behavior of dynamic systems 5. In this approach, the ODE that defines the time derivative of the system output is expressed as a deep neural network; when provided with initial conditions, an ODE solver determines the system response at future times. Chen et al. 6 extended this approach by expressing hidden variables that are difficult to probe with higher-order derivatives of the output, which are then replaced with mathematically equivalent time-delayed versions. External inputs can be applied to the network in a similar time-delayed fashion.
In this work, DW motion by spin-transfer torque excitation is modeled utilizing the neural ODE framework. Temporal DW position data along a magnetic track excited by a set of random sinusoidal current inputs are generated by mumax3 7 and are used to train a neural ODE in a supervised fashion through backpropagation. For a different set of random sinusoidal current inputs, the DW position forecasted by the trained network matches with the results generated by mumax3 with very high accuracy (Fig. 2) while reducing the simulation time by a factor greater than 200x, thereby opening the path to efficiently design integrated systems interconnecting a large number of DWMTJs.
References
1 M. Alamdar, T. Leonard, C. Cui et al., Appl. Phys. Lett., vol. 118, no. 11, p. 112401 (2021).
2 N. Hassan, X. Hu, L. Jiang-Wei et al., Jour. Appl. Phys., vol. 124, no. 15, p. 152127 (2018).
3 W. H. Brigner, N. Hassan, L. Jiang-Wei et al., IEEE Trans. Electron Devices, vol. 66, no. 11, pp. 4970-4975 (2019).
4 X. Hu, A. Timm, W. H. Brigner et al., IEEE Trans. Electron Devices, vol. 66, no. 6, pp. 2817-2821 (2019).
5 R. T. Q. Chen, Y. Rubanova, J. Bettencourtet al., Proceedings of NeurIPS, 31 (2018).
6 X. Chen, F. A. Araujo, M. Riou et al, Nat. Comm., vol. 12, no. 1016 (2022).
7 A. Vansteenkiste, J. Leliaert, M. Dvornik et al., AIP Advances, vol. 4, no. 10, p. 107133 (2014).
Up (blue) and down (red) polarized magnetic domains along a track separated by a DW (yellow). Current inputs control the position of the DW by applying spin-transfer torque.
DW position forecasted by a trained neural ODE in comparison with mumax3 simulation.