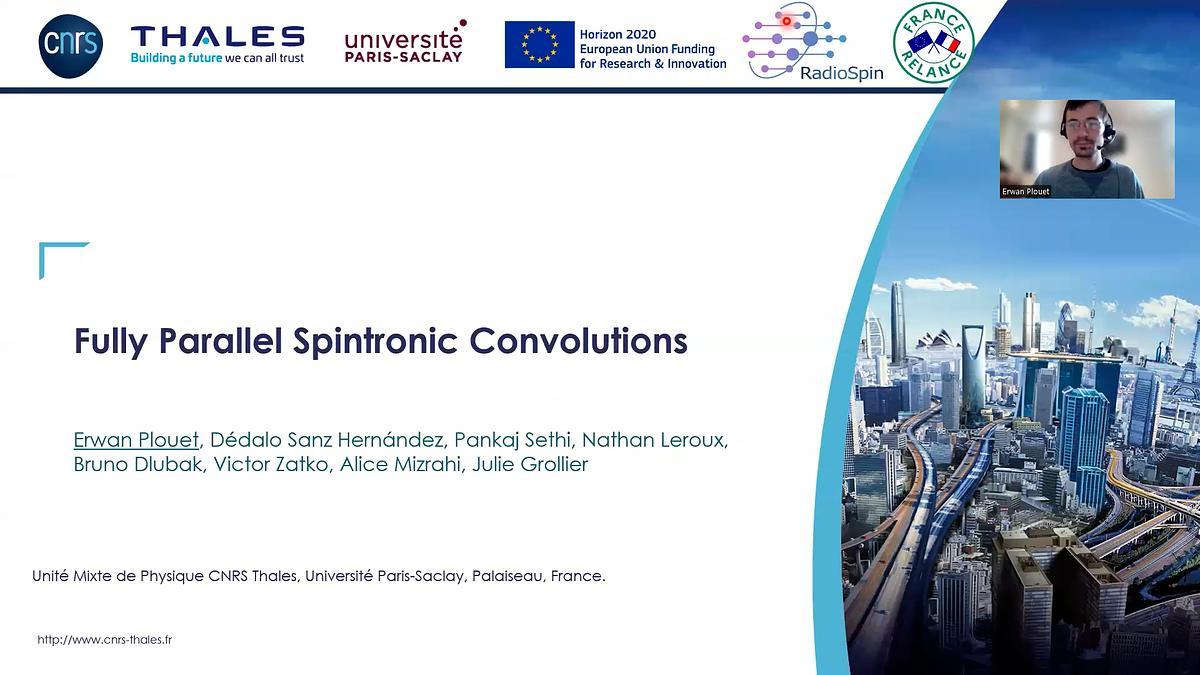
Premium content
Access to this content requires a subscription. You must be a premium user to view this content.
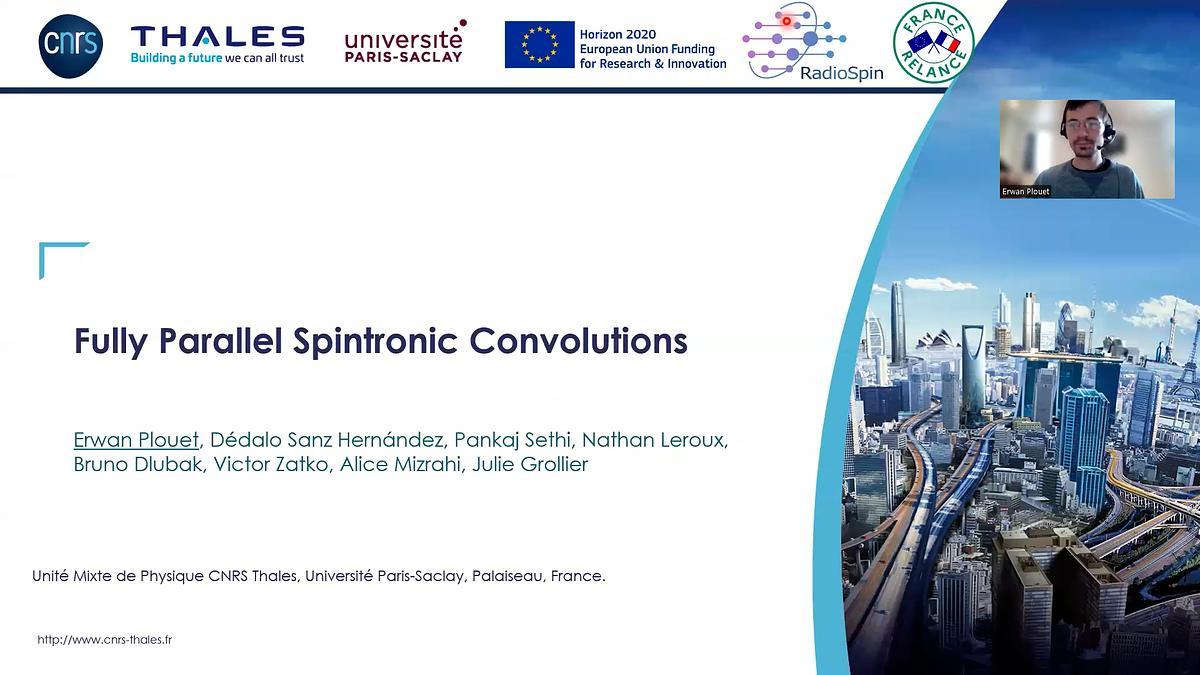
technical paper
Fully Parallel Convolution with Chains of Spin
Convolutional neural networks (CNN) are state of the art algorithms for image processing. Despite a small number of synaptic weights, the CNNs remain computationally costly to train in software due to the necessity to exchange huge data flows between memory and processing units. Unconventional hardware is suited to address this limitation.
In neuromorphic spintronics, it has been previously demonstrated that the spin diode effect can be used to apply a synaptic weight on a radiofrequency signal 1. Here we go a step beyond, to show an experimental implementation of a convolutional layer employing chains of spin-diodes. We design a compact architecture of 3 chains with 3 spin-diodes connected in series, that performs a padded convolution with a filter of 3 pixels on 5 input pixels multiplexed in frequency in one RF signal (See Figure 1). The synaptic weights corresponding to the filter are encoded by a small frequency detuning between the inputs and the spin-diodes resonance and are controlled in parallel using currents in 3 strip lines.
This architecture exploits the intrinsic weight redundancy of convolutions (all filters share the same weights) to enhance the compacity of the hardware and greatly simplify the process of updating a weight, only requiring updating a the current in one strip line.
We will also present processed experimental results highlighting the scalability of the proposed architecture. An error on the performed convolution as low as 0.28% was achieved (See Figure 2).
The proposed architecture enables us to both reduce the size of this hardware implementation while, at the same time, performing the convolution in one timestep contrary to previous time-multiplexed implementations. According to a previous study 3 we can achieve to decrease by one order of magnitude the energy consumption compared to current GPUs and two orders of magnitude in operating latency. This proof of concept of spintronic CNN, opens the path to spintronic neural networks that can exploit the power of convolutional layers in a fully parallel, compact, and energy efficient way.