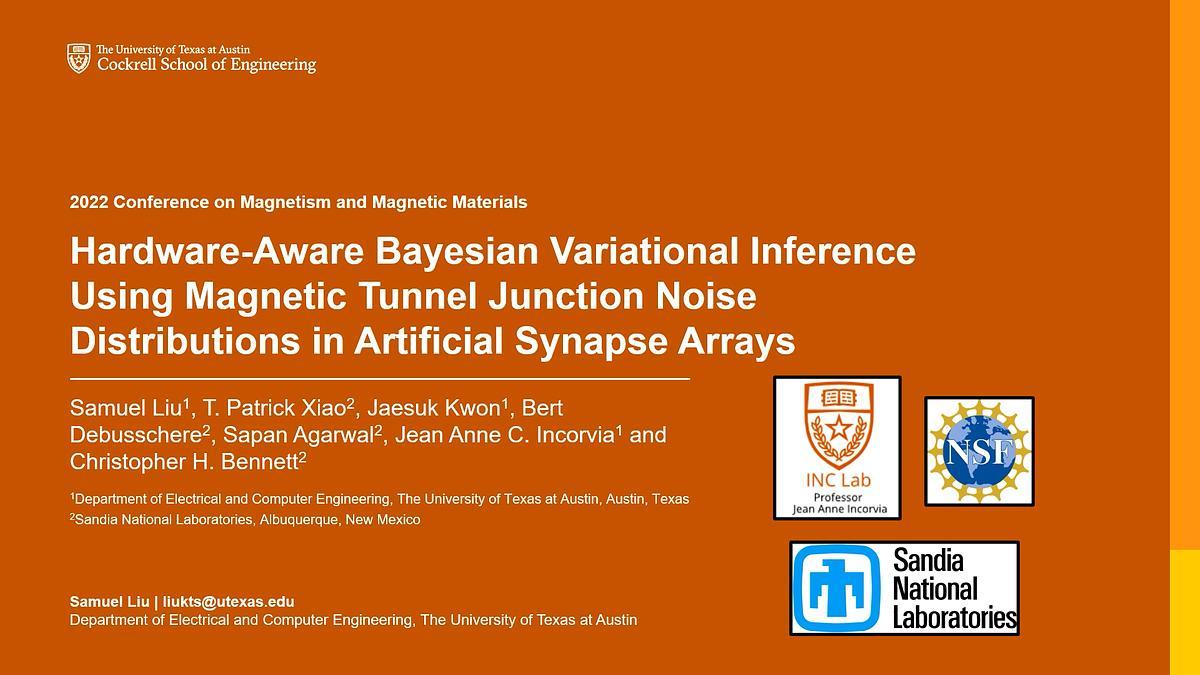
Premium content
Access to this content requires a subscription. You must be a premium user to view this content.
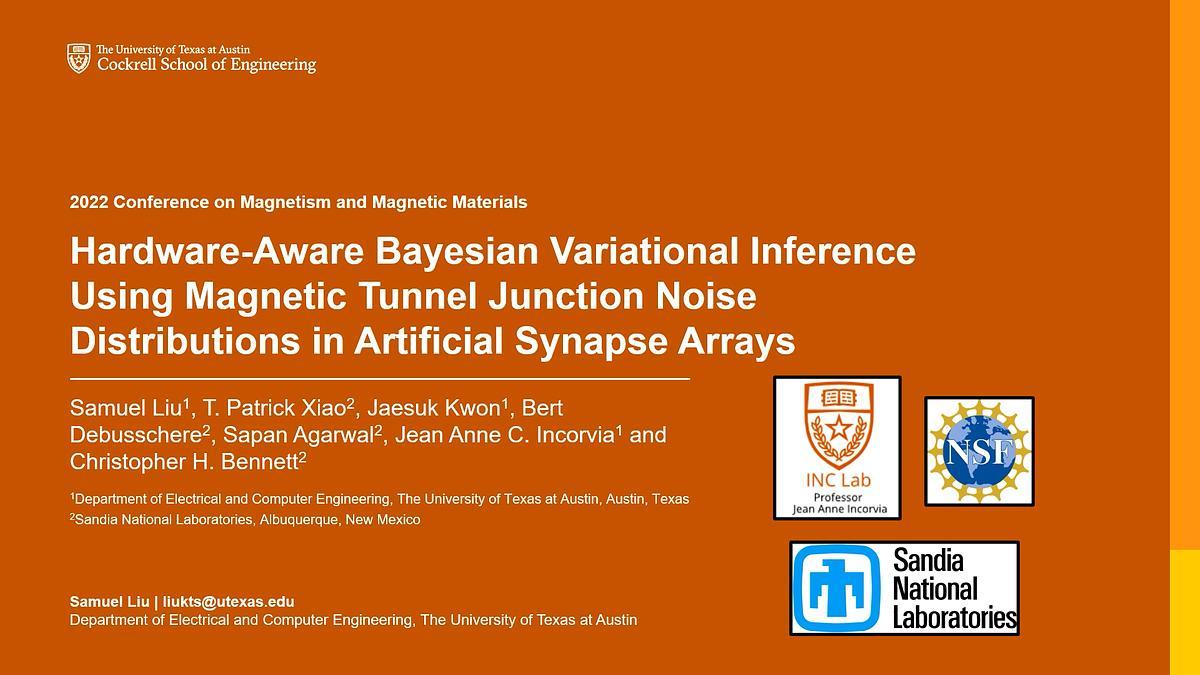
technical paper
Hardware Aware Bayesian Variational Inference Using Magnetic Tunnel Junction Noise Distributions in Artificial Synapse Arrays
Artificial neural networks (ANNs) are powerful tools for data intensive classification and regression, but the memory wall in von Neumann architectures prevents efficient processing 1. Though there are many candidates for devices and architectures to realize ANNs, there are no comprehensive solutions to implement hardware Bayesian NNs (BNNs). BNNs are much less susceptible to overfitting when available data is sparse or unreliable, accomplished by representing and learning the weights of the network as distributions instead of deterministic values 2. We propose a novel spintronic synaptic cell design that can implement tunable distributions by taking advantage of the intrinsic stochasticity of magnetic materials.
The design of the cell is composed of a noise and weight encoding element respectively (see Fig. 1). The noisy element is a circular in-plane magnetic tunnel junction (MTJ) that relies on thermal activation to output a random conductance within the range of its TMR. This TMR can then be controlled through several methods such as voltage-controlled magnetic anisotropy (VCMA) 3 or magneto-ionics 4. Combined with a weight encoding conductance element like a domain wall-magnetic tunnel junction (DW-MTJ) artificial synapse 5,6, we propose a novel cell design that can represent the distributions necessary for inference in a hardware BNN. We demonstrate device functionality through Landau-Lifshitz-Gilbert and micromagnetics 7 models, model analog Bayesian inference in CrossSim 8 for regression (see Fig. 2) and classification to detect out-of-distribution data, and compare the system’s energy efficiency to CMOS implementations. These results propose a novel synapse implementation for accurate and efficient Bayesian inference, a foundational step toward hardware BNN accelerators.
SNL is managed and operated by NTESS under DOE NNSA contract DE-NA0003525.