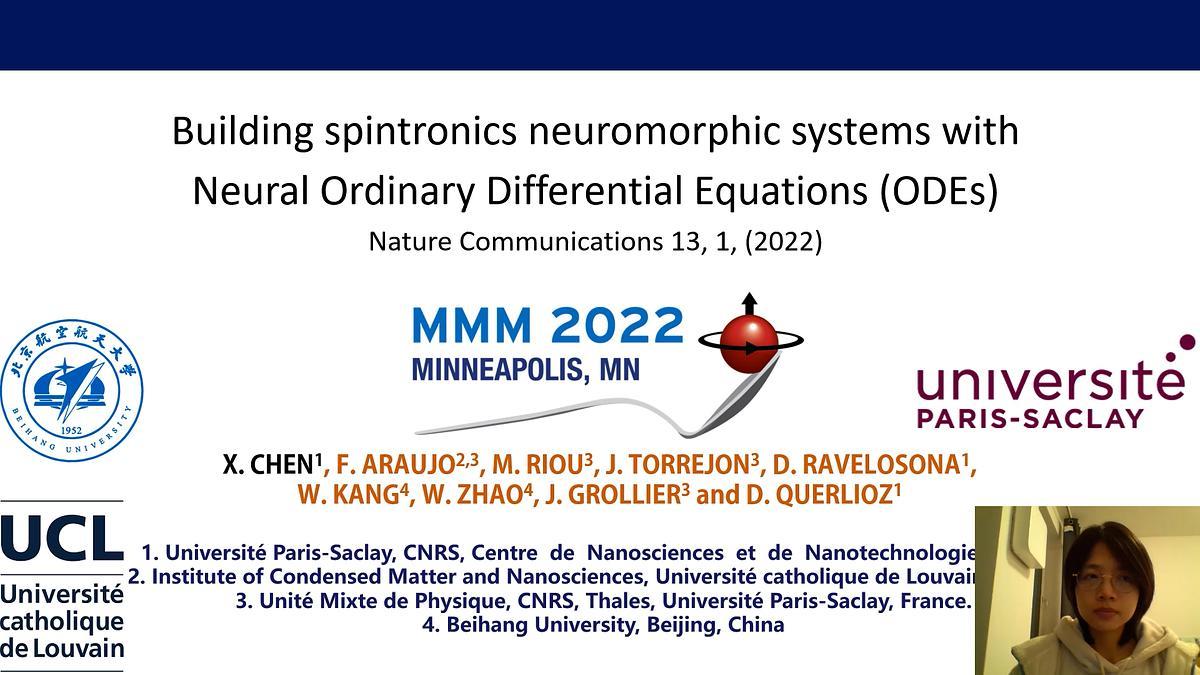
Premium content
Access to this content requires a subscription. You must be a premium user to view this content.
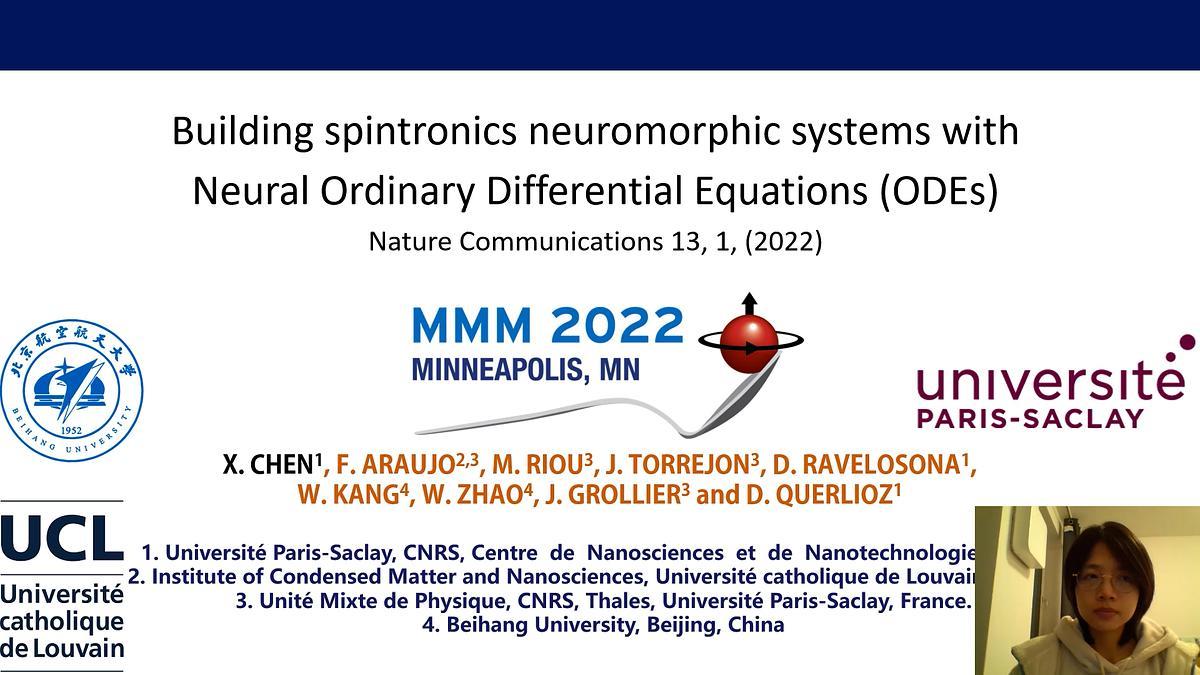
technical paper
Building spintronics neuromorphic systems with Neural Ordinary Differential Equations
Neuromorphic computing is a particularly exciting application of spintronics, able to exploit its rich physics to achieve low-power-consumption computation inspired by the brain. Designing neuromorphic systems requires simulating the response of spintronic devices to multiple types of inputs over long time periods. Currently, the dominant approach to predicting the complex behavior of spintronic devices is micromagnetic simulation. This technique, however, requires very long simulation times, easily reaching weeks in time-dependent experiments or in micrometer-scale devices.
In this work, we take an alternative road by adopting a deep learning technique, Neural Ordinary Differential Equations (ODEs), to model spintronic devices and develop spintronic systems. We show that Neural ODEs, trained on a minimal amount of data, can predict the behavior of spintronic devices with high accuracy and an extremely efficient simulation time, compared to micromagnetic simulations. For this purpose, we re-frame the formalism of Neural Ordinary Differential Equations (ODEs) 1 to the constraints of spintronics: few measured outputs, multiple inputs and internal parameters (Fig. 1). We demonstrate with Neural ODEs an acceleration factor over 200 compared to micromagnetic simulations for a neuromorphic system -- a reservoir computer made of magnetic skyrmions (20 minutes compared to three days). We also demonstrate that this state-of-the-art deep learning technique for time series modeling can be applied to model an experimental neuromorphic experiment 2, in which spin-torque nano-oscillators are used as reservoir computers to achieve a spoken digit recognition task (Fig. 2). Neural ODEs can therefore constitute a disruptive tool for developing spintronic applications in complement to micromagnetic simulations, which are time-consuming and cannot fit experiments when noise or imperfections are present 3.