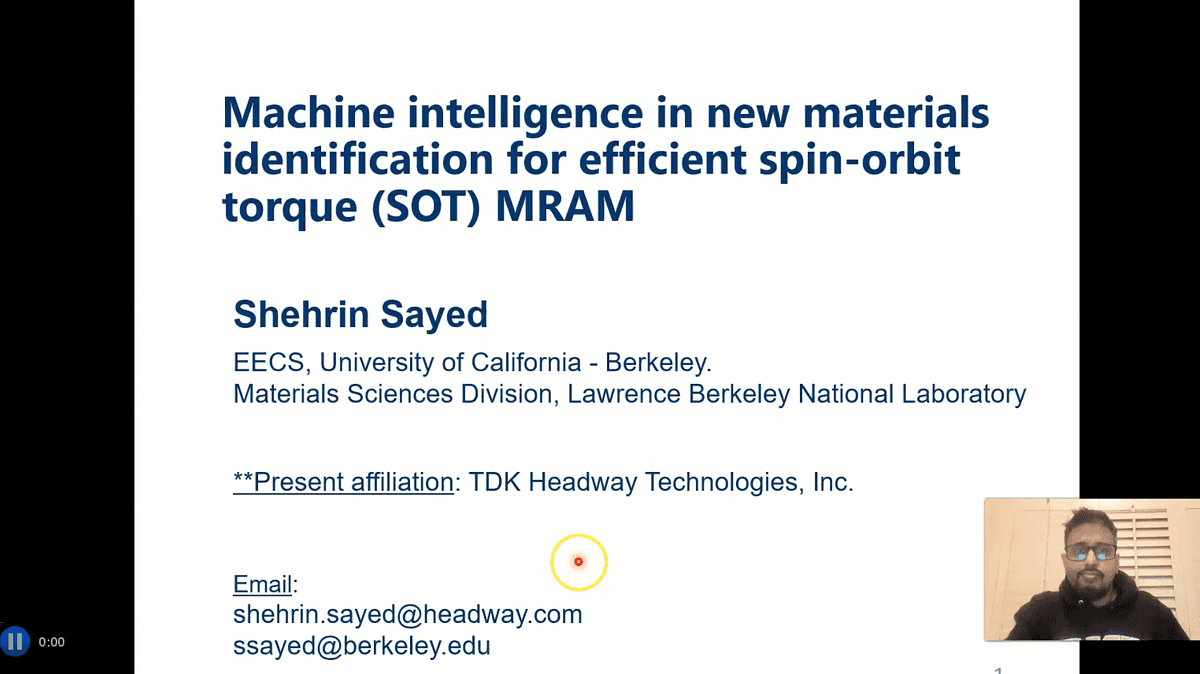
Premium content
Access to this content requires a subscription. You must be a premium user to view this content.
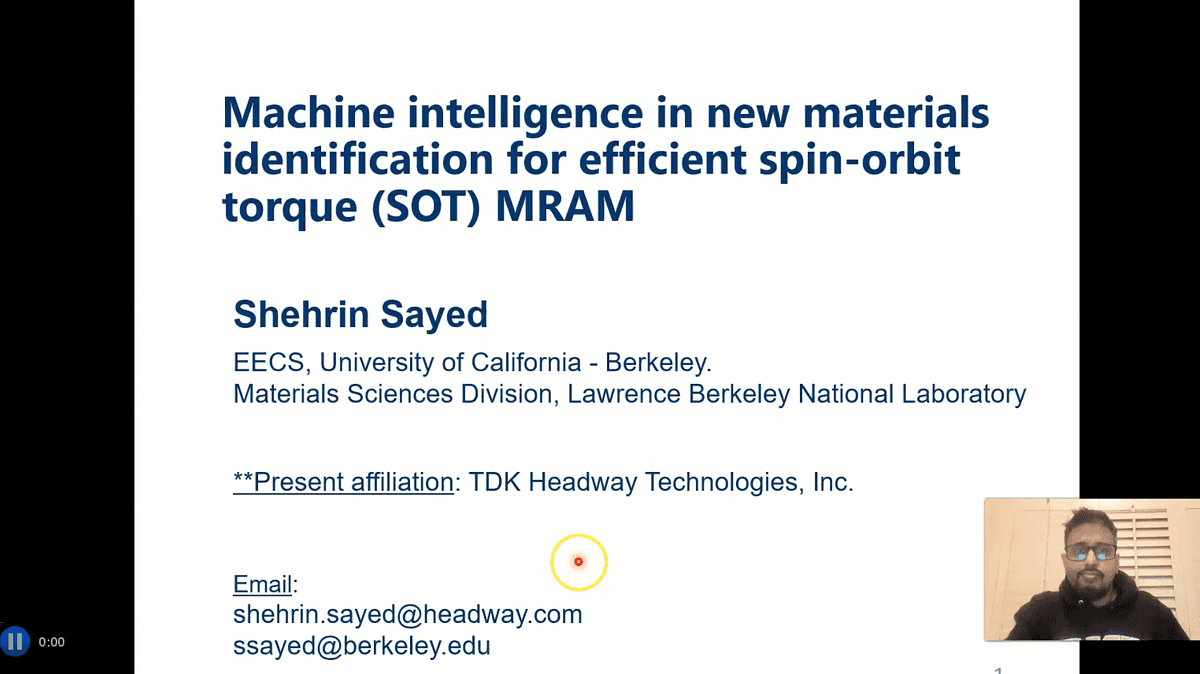
technical paper
Machine intelligence in new materials identification for efficient spin orbit torque (SOT) MRAM.
There is a growing interest in materials exhibiting large spin-orbit torques (SOT) to achieve significant improvements in magnetoresistive random access memory (MRAM) technology 1. It is well-recognized that materials with SOT ratios much greater than unity are needed to bring energy efficiency in SOT MRAM; however, only a handful of such materials have been identified using conventional theoretical and experimental methodologies. Thus, understanding the physics and materials for large SOT is a topic of ongoing research 1-2.
In this talk, I will discuss a new approach using machine intelligence to quantitatively predict new materials with large SOT ratios. I'll show that a machine can learn special concepts in materials sciences, physics, and engineering by reading the literature. Such a "well-trained" machine can identify patterns hidden within a large body of literature and quantitatively relate materials to the phenomenon of "spin-orbit torque."
We use a word embedding model 3 to train a neural network with a collection of unlabeled abstract text from various scientific journals relevant to our work. The word embedding model represents words in the text corpus into high-dimensional vectors, and the relationship among these vectors exhibits knowledge in magnetism and spintronics. A correlation pattern among various vectors representing materials names and the word "spin-orbit torque" quantitatively project spin Hall conductivity and SOT factor for corresponding materials. The projected numbers for known materials agree with known experimental reports. Such quantitative agreement is non-trivial as the machine does not learn numerical values reported in the literature, but the patterns are obtained based on the context in which the words appeared in the text corpus.
Surprisingly, our machine intelligence-based approach predicted 32 new materials to exhibit SOT, which have not been discussed yet in the context of SOT to our knowledge. Interestingly, 9 of these predicted materials are expected to show a SOT factor much greater than unity, with the largest expected value of 36.4. One of them quantitatively matched our independent experiment on a silicide.
References 1 IEEE Trans. Magnetics 57(7), 1-39 (2021).
2 Phys. Rev. Applied 15, 054004 (2021).
3 Nature 571, 95-98 (2019).