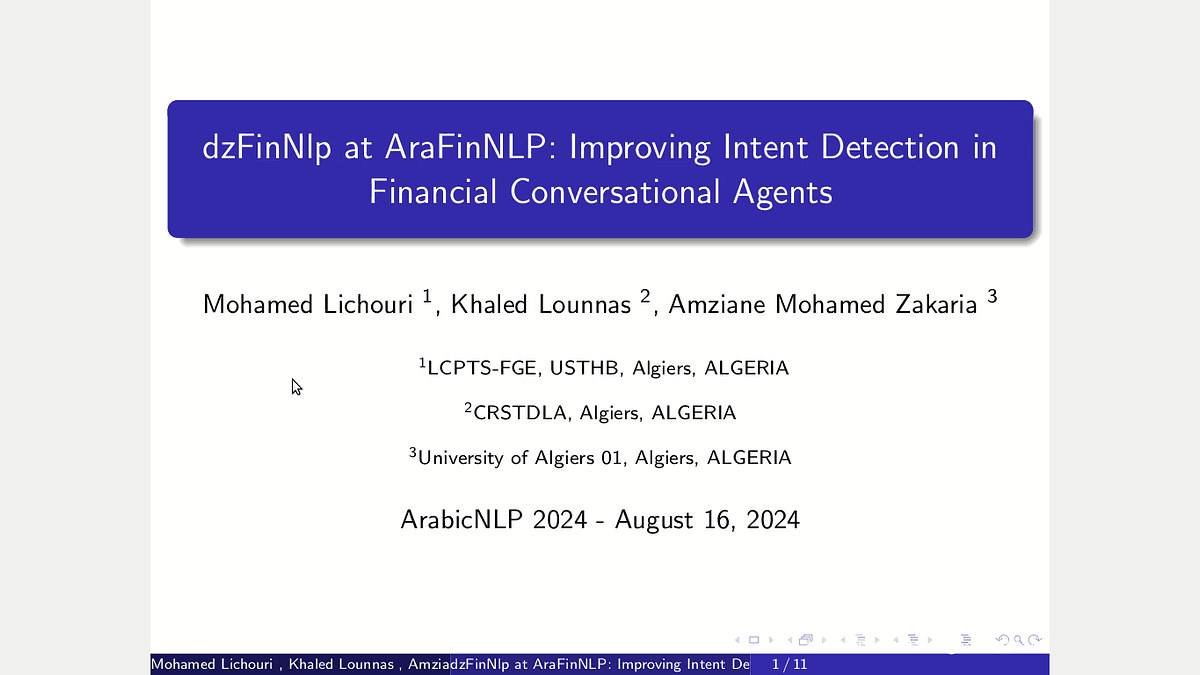
Premium content
Access to this content requires a subscription. You must be a premium user to view this content.
Monthly subscription - $9.99Pay per view - $4.99Access through your institutionLogin with Underline account
Need help?
Contact us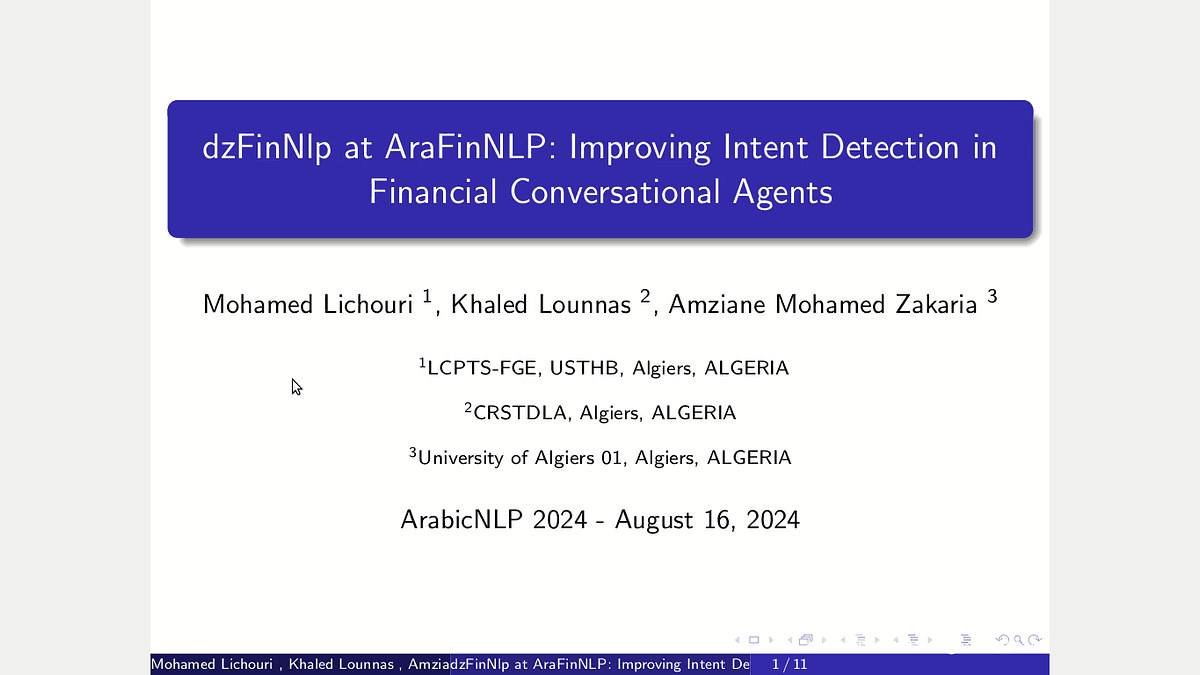
UNDERLINE DOI: https://doi.org/10.48448/efp7-8x81
workshop paper