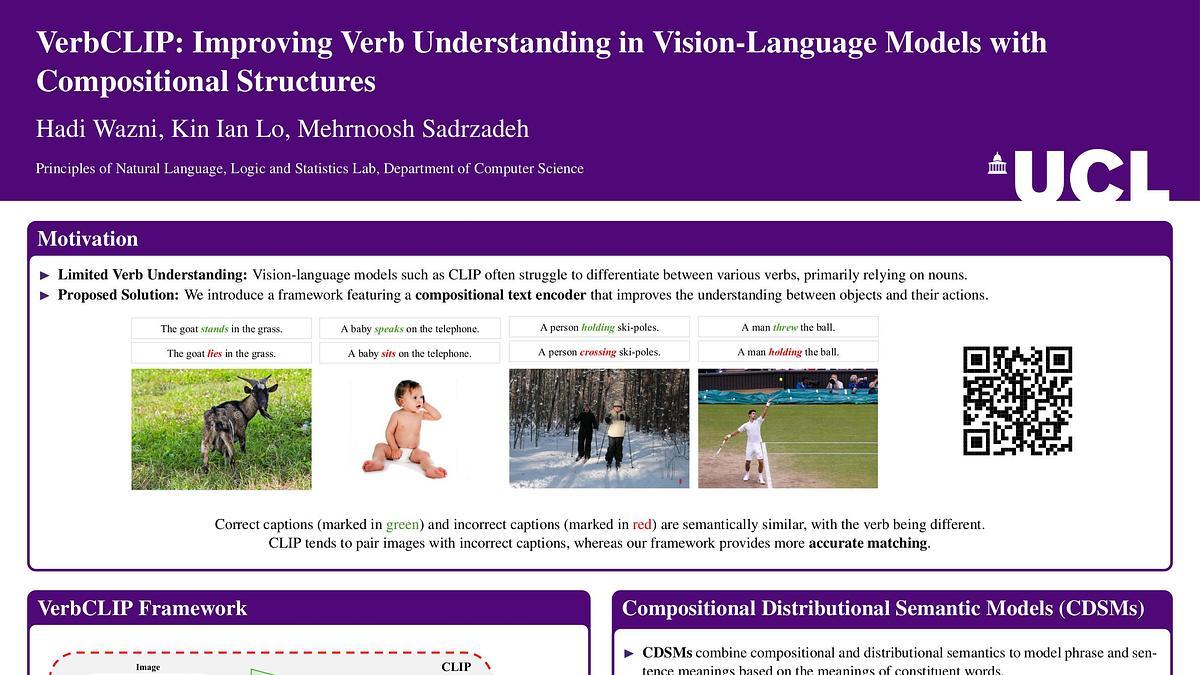
Premium content
Access to this content requires a subscription. You must be a premium user to view this content.
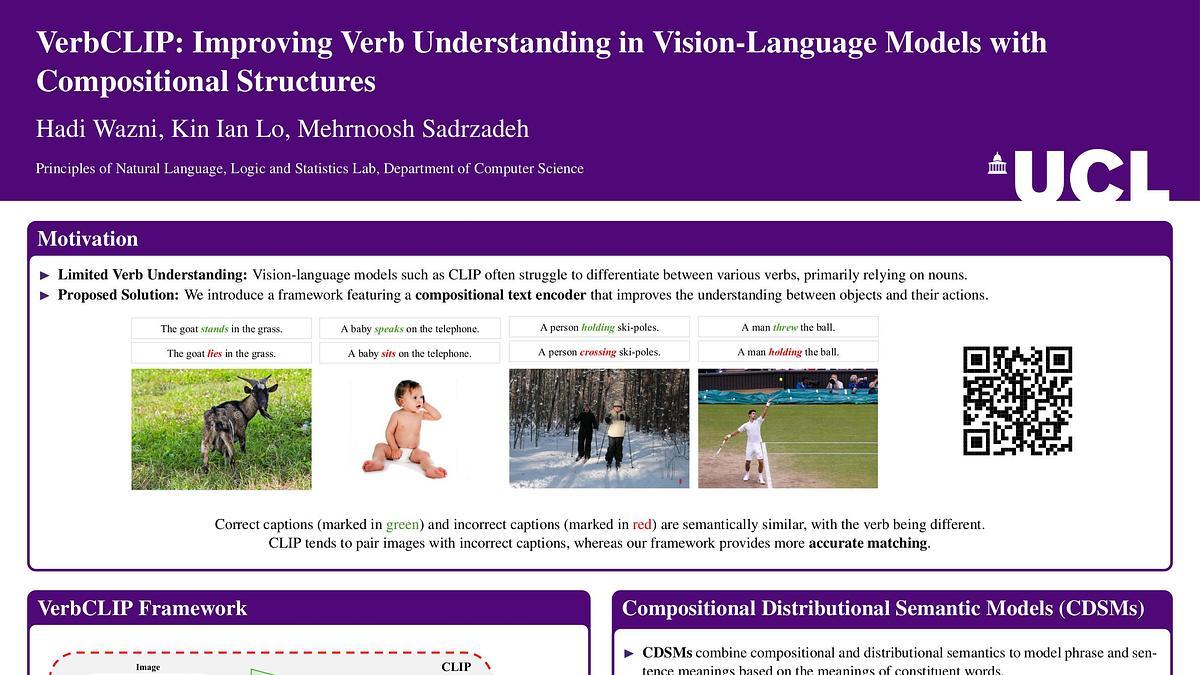
workshop paper
VerbCLIP: Improving Verb Understanding in Vision-Language Models
keywords:
grammatical structure
visual-language
categorial grammar
compositional distributional semantics
clip
alignment
transformers
Verbs describe the dynamics of interactions between people, objects, and their environments. They play a crucial role in language formation and understanding. Nonetheless, recent vision-language models like CLIP predominantly rely on nouns and have a limited account of verbs. This limitation affects their performance in tasks requiring action recognition and scene understanding. In this work, we introduce VerbCLIP, a verb-centric vision-language model which learns meanings of verbs based on a compositional approach to statistical machine learning. Our methods significantly outperform CLIP in zero-shot performance on the VALSE, VL-Checklist, and SVO-Probes datasets, with improvements of +2.38\%, +3.14\%, and +1.47\%, without fine-tuning. Fine-tuning resulted in further improvements, with gains of +2.85\% and +9.2\% on the VALSE and VL-Checklist datasets.