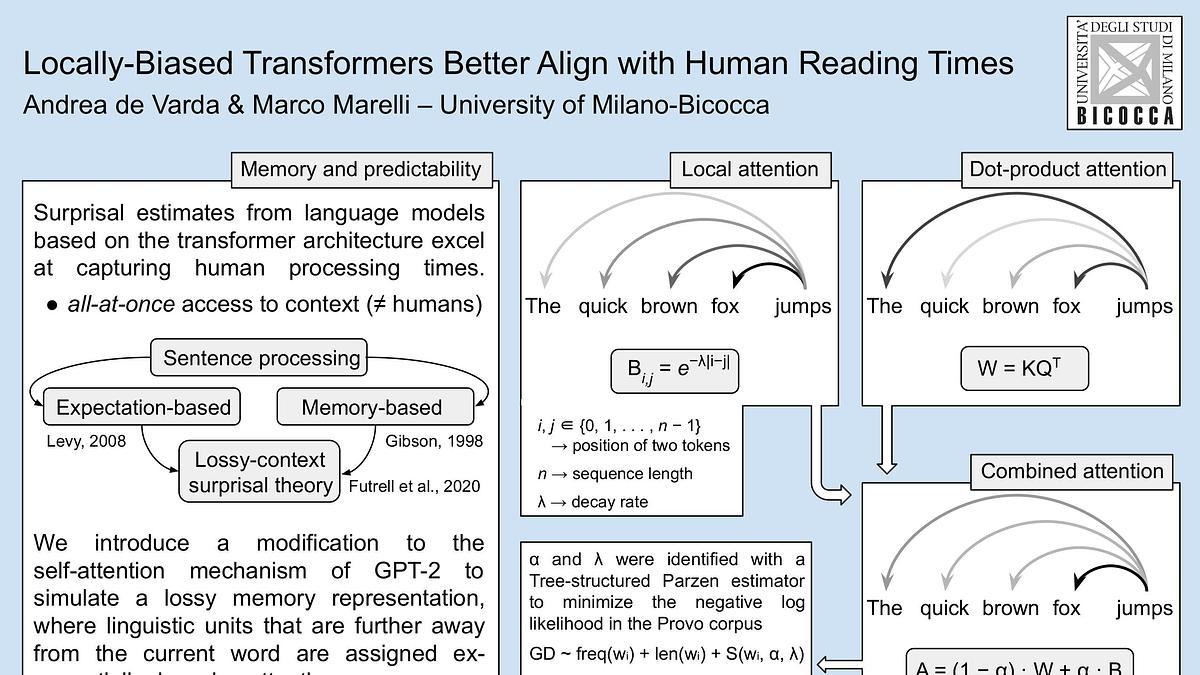
Premium content
Access to this content requires a subscription. You must be a premium user to view this content.
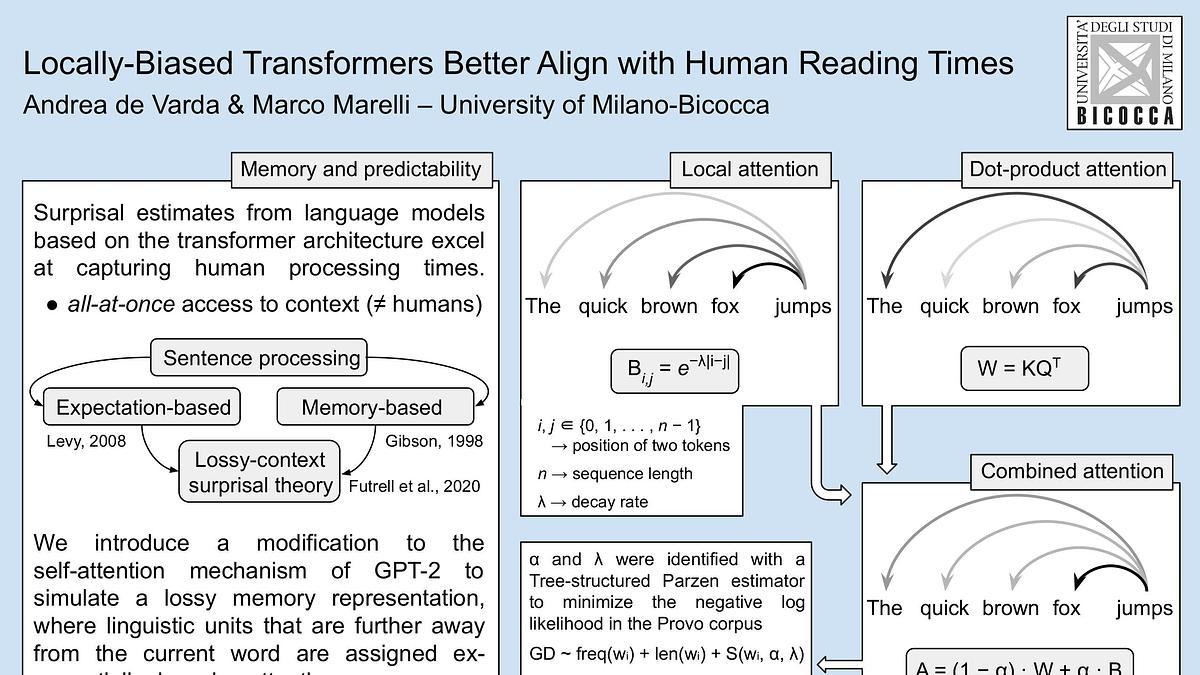
workshop paper
Locally Biased Transformers Better Align with Human Reading Times
keywords:
lossy-context surprisal theory
predictability
working memory
transformer models
Recent psycholinguistic theories emphasize the interdependence between linguistic expectations and memory limitations in human language processing. We modify the self-attention mechanism of a transformer model to simulate a lossy context representation, biasing the model's predictions to give additional weight to the local linguistic context. We show that surprisal estimates from our locally-biased model generally provide a better fit to human psychometric data, underscoring the sensitivity of the human parser to local linguistic information.