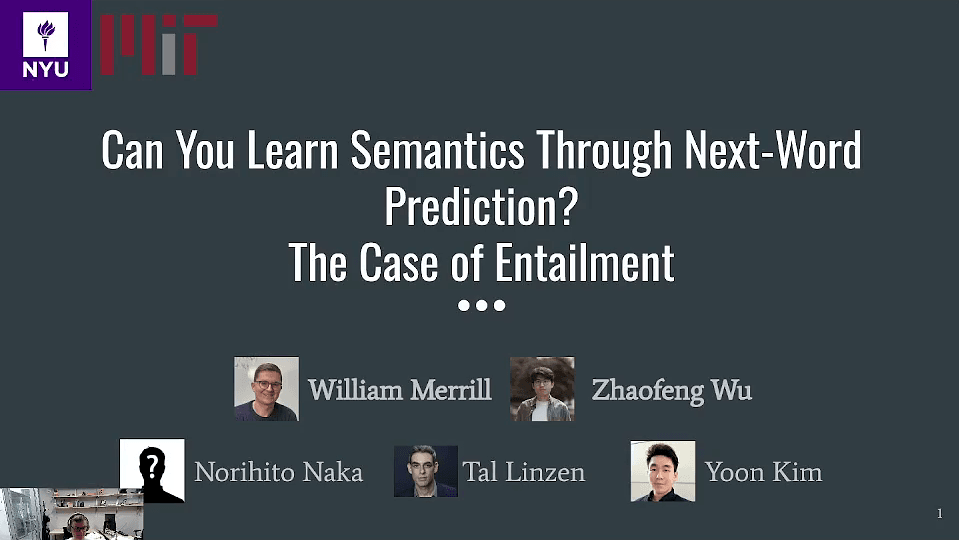
Premium content
Access to this content requires a subscription. You must be a premium user to view this content.
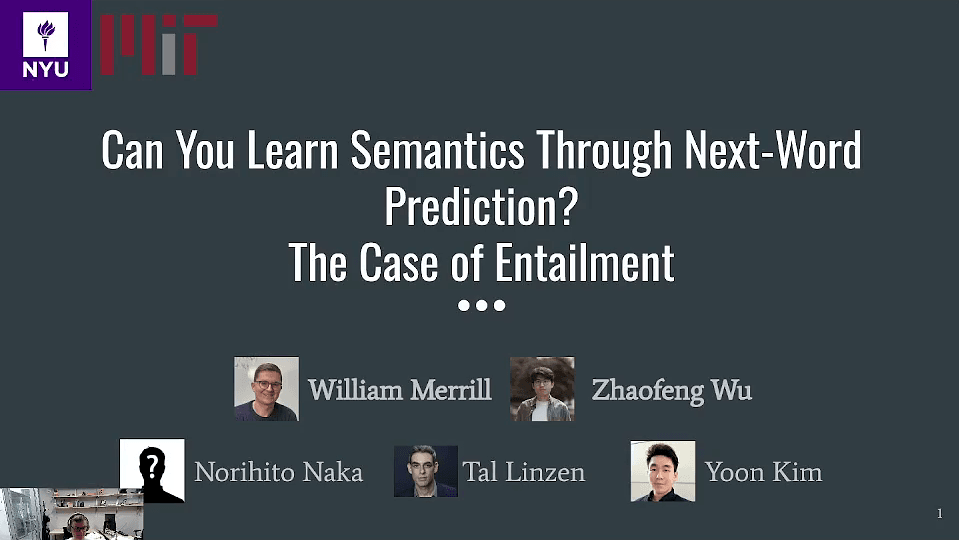
poster
Can You Learn Semantics Through Next-Word Prediction? The Case of Entailment
keywords:
distributional semantics
entailment
language models
Do LMs infer the semantics of text from co-occurrence patterns in their training data? Merrill et al. (2022) argue that, in theory, sentence co-occurrence probabilities predicted by an optimal LM should reflect the entailment relationship of the constituent sentences, but it is unclear whether probabilities predicted by neural LMs encode entailment in this way because of strong assumptions made by Merrill et al. (namely, that humans always avoid redundancy). In this work, we investigate whether their theory can be used to decode entailment relations from neural LMs. We find that a test similar to theirs can decode entailment relations between natural sentences, well above random chance, though not perfectly, across many datasets and LMs. This suggests LMs implicitly model aspects of semantics to predict semantic effects on sentence co-occurrence patterns. However, we find the test that predicts entailment in practice works in the opposite direction to the theoretical test. We thus revisit the assumptions underlying the original test, finding its derivation did not adequately account for redundancy in human-written text. We argue that better accounting for redundancy related to explanations might derive the observed flipped test and, more generally, improve computational models of speakers in linguistics.