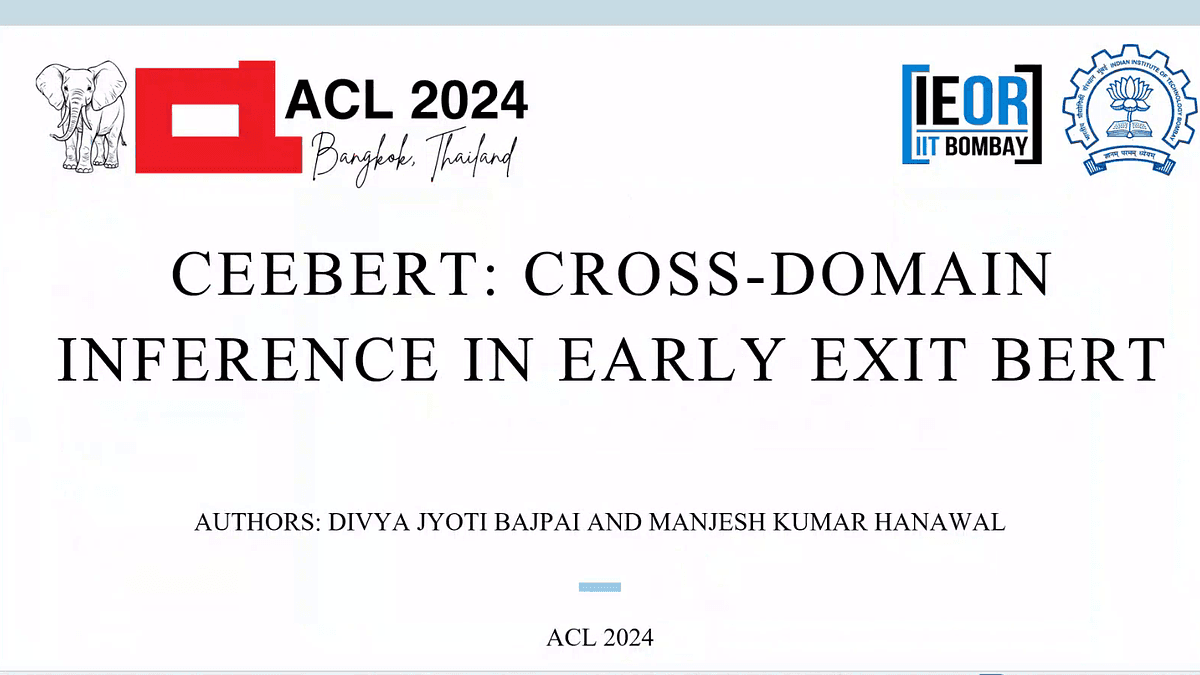
Premium content
Access to this content requires a subscription. You must be a premium user to view this content.
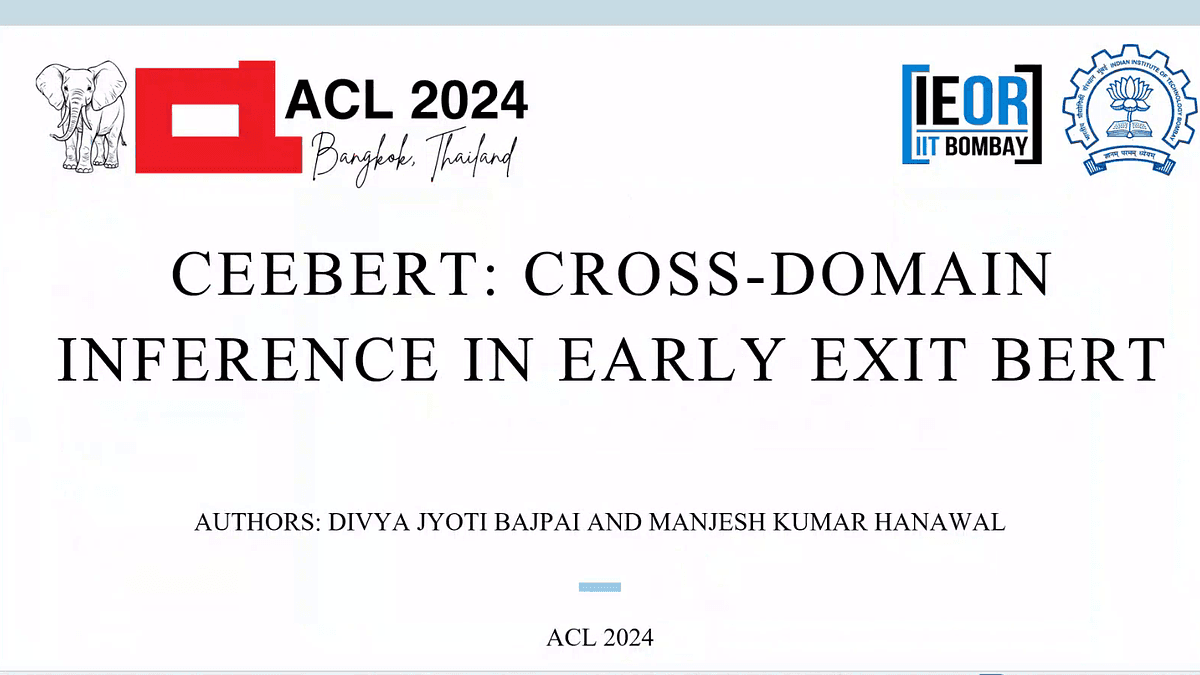
poster
CeeBERT: Cross-Domain Inference in Early Exit BERT
keywords:
cross-domain inference
pre-trained language models
bert
Pre-trained Language Models (PLMs), like BERT, with self-supervision objectives exhibit remarkable performance and generalization across various tasks. However, they suffer in inference latency due to their large size. To address this issue, side branches are attached at intermediate layers, enabling early inference of samples without requiring them to pass through all layers. However, the challenge is to decide which layer to infer and exit each sample so that the accuracy and latency are balanced. Moreover, the distribution of the samples to be inferred may differ from that used for training necessitating cross-domain adaptation. We propose an online learning algorithm named Cross-Domain Inference in Early Exit BERT (CeeBERT) that dynamically determines early exits of samples based on the level of confidence at each exit point. CeeBERT learns optimal thresholds from domain-specific confidence observed at intermediate layers on the fly, eliminating the need for labeled data. Experimental results on five distinct datasets with BERT and ALBERT models demonstrate CeeBERT's ability to improve latency by reducing unnecessary computations with minimal drop in performance. By adapting to the threshold values, CeeBERT can speed up the BERT/ALBERT models by $2\times$ - $3.1\times$ with minimal drop in accuracy. The anonymized source code is available at https://github.com/Div290/CeeBERT.