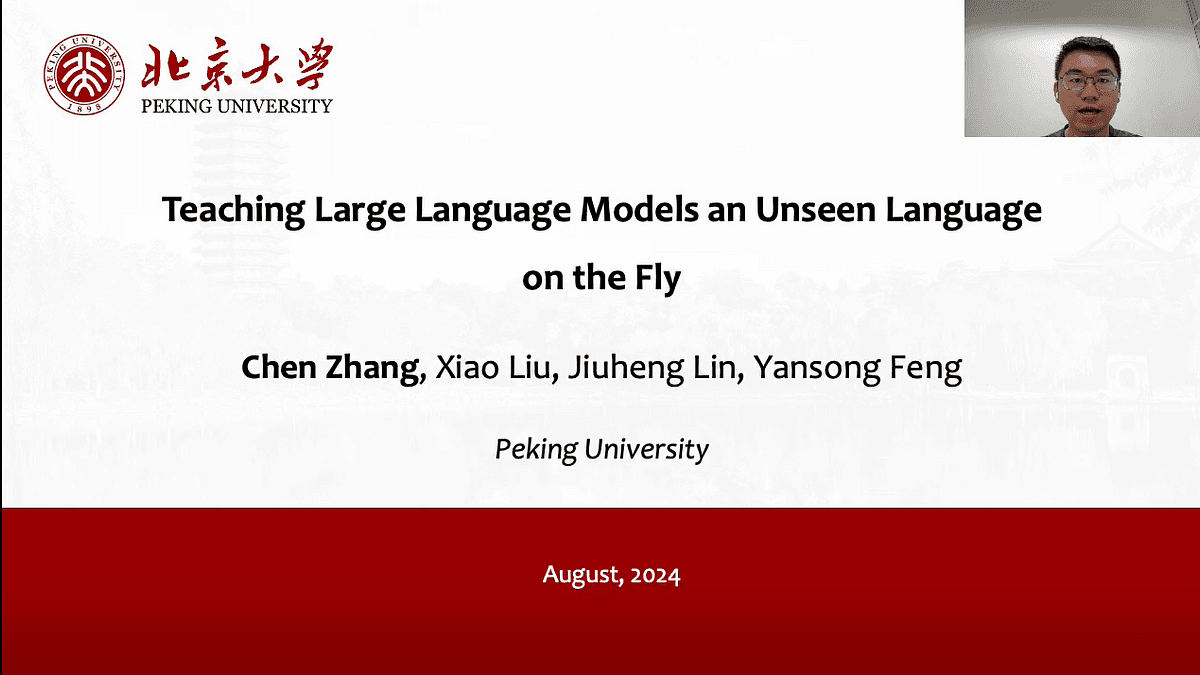
Premium content
Access to this content requires a subscription. You must be a premium user to view this content.
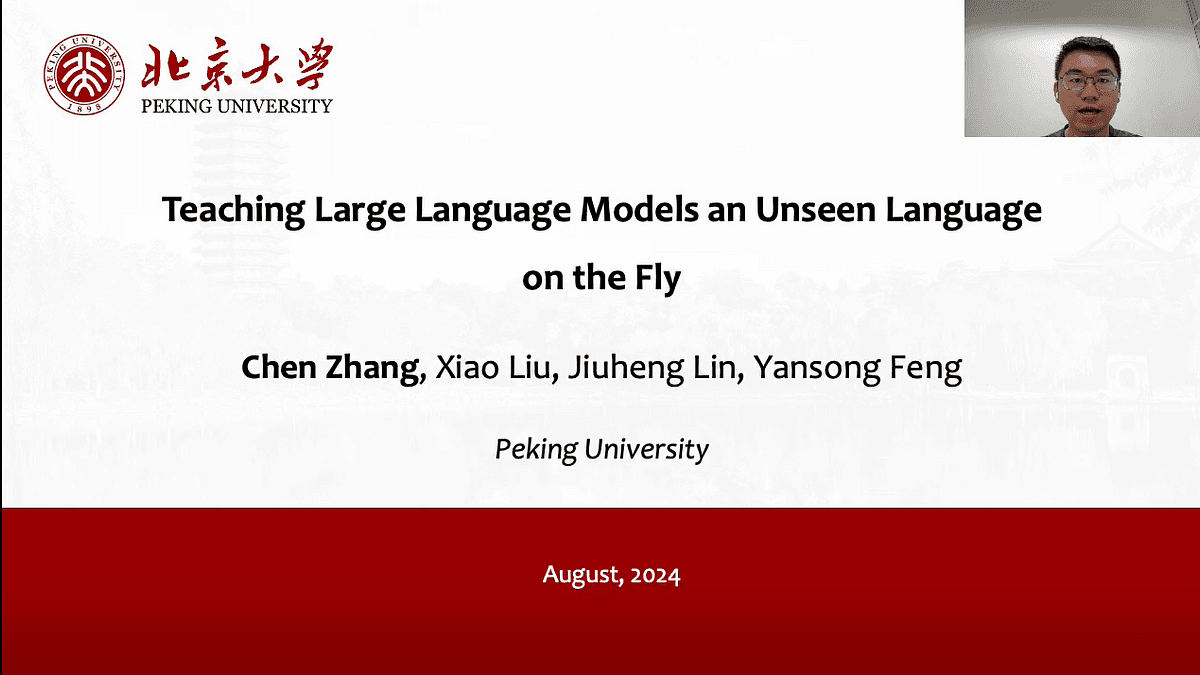
poster
Teaching Large Language Models an Unseen Language on the Fly
keywords:
linguistic diversity
low-resource languages
large language models
Existing large language models struggle to support numerous low-resource languages, particularly the extremely low-resource ones, for which there is minimal training data available for effective parameter updating. We thus investigate whether LLMs can learn a new language on the fly solely through prompting. To study this question, we collect a research suite for Zhuang, a language supported by no LLMs currently. We introduce DiPMT++, a framework for adapting LLMs to unseen languages by in-context learning. Using a dictionary and 5K parallel sentences only, DiPMT++ significantly enhances the performance of GPT-4 from 0 to 16 BLEU for Chinese-to-Zhuang translation and achieves 32 BLEU for Zhuang-to-Chinese translation. We also validate the effectiveness of our framework on Kalamang, another unseen language. Furthermore, we demonstrate the practical utility of DiPMT++ in aiding humans in translating completely unseen languages, which could contribute to the preservation of linguistic diversity.